Tech
A human-centric approach to adopting AI
Published
10 months agoon
By
Terry Power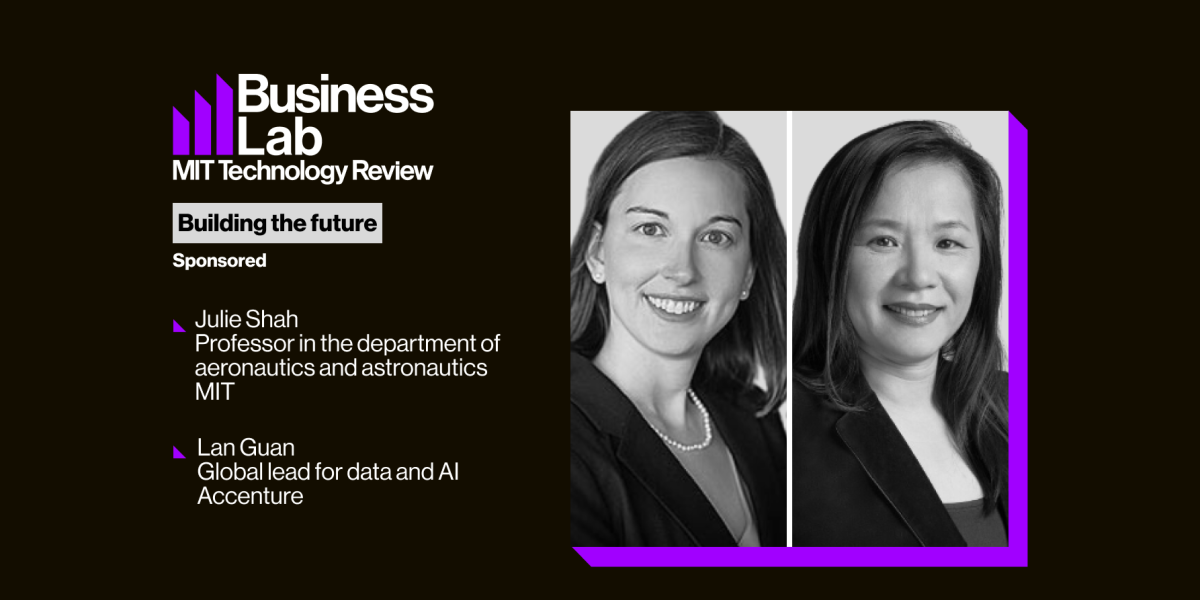
So very quickly, I gave you examples of how AI has become pervasive and very autonomous across multiple industries. This is a kind of trend that I am super excited about because I believe this brings enormous opportunities for us to help businesses across different industries to get more value out of this amazing technology.
Laurel: Julie, your research focuses on that robotic side of AI, specifically building robots that work alongside humans in various fields like manufacturing, healthcare, and space exploration. How do you see robots helping with those dangerous and dirty jobs?
Julie: Yeah, that’s right. So, I’m an AI researcher at MIT in the Computer Science & Artificial Intelligence Laboratory (CSAIL), and I run a robotics lab. The vision for my lab’s work is to make machines, these include robots. So computers become smarter, more capable of collaborating with people where the intention is to be able to augment rather than replace human capability. And so we focus on developing and deploying AI-enabled robots that are capable of collaborating with people in physical environments, working alongside people in factories to help build planes and build cars. We also work in intelligent decision support to support expert decision makers doing very, very challenging tasks, tasks that many of us would never be good at no matter how long we spent trying to train up in the role. So, for example, supporting nurses and doctors and running hospital units, supporting fighter pilots to do mission planning.
The vision here is to be able to move out of this sort of prior paradigm. In robotics, you could think of it as… I think of it as sort of “era one” of robotics where we deployed robots, say in factories, but they were largely behind cages and we had to very precisely structure the work for the robot. Then we’ve been able to move into this next era where we can remove the cages around these robots and they can maneuver in the same environment more safely, do work in the same environment outside of the cages in proximity to people. But ultimately, these systems are essentially staying out of the way of people and are thus limited in the value that they can provide.
You see similar trends with AI, so with machine learning in particular. The ways that you structure the environment for the machine are not necessarily physical ways the way you would with a cage or with setting up fixtures for a robot. But the process of collecting large amounts of data on a task or a process and developing, say a predictor from that or a decision-making system from that, really does require that when you deploy that system, the environments you’re deploying it in look substantially similar, but are not out of distribution from the data that you’ve collected. And by and large, machine learning and AI has previously been developed to solve very specific tasks, not to do sort of the whole jobs of people, and to do those tasks in ways that make it very difficult for these systems to work interdependently with people.
So the technologies my lab develops both on the robot side and on the AI side are aimed at enabling high performance and tasks with robotics and AI, say increasing productivity, increasing quality of work, while also enabling greater flexibility and greater engagement from human experts and human decision makers. That requires rethinking about how we draw inputs and leverage, how people structure the world for machines from these sort of prior paradigms involving collecting large amounts of data, involving fixturing and structuring the environment to really developing systems that are much more interactive and collaborative, enable people with domain expertise to be able to communicate and translate their knowledge and information more directly to and from machines. And that is a very exciting direction.
It’s different than developing AI robotics to replace work that’s being done by people. It’s really thinking about the redesign of that work. This is something my colleague and collaborator at MIT, Ben Armstrong and I, we call positive-sum automation. So how you shape technologies to be able to achieve high productivity, quality, other traditional metrics while also realizing high flexibility and centering the human’s role as a part of that work process.
Laurel: Yeah, Lan, that’s really specific and also interesting and plays on what you were just talking about earlier, which is how clients are thinking about manufacturing and AI with a great example about factories and also this idea that perhaps robots aren’t here for just one purpose. They can be multi-functional, but at the same time they can’t do a human’s job. So how do you look at manufacturing and AI as these possibilities come toward us?
Lan: Sure, sure. I love what Julie was describing as a positive sum gain of this is exactly how we view the holistic impact of AI, robotics type of technology in asset-heavy industries like manufacturing. So, although I’m not a deep robotic specialist like Julie, but I’ve been delving into this area more from an industry applications perspective because I personally was intrigued by the amount of data that is sitting around in what I call asset-heavy industries, the amount of data in IoT devices, right? Sensors, machines, and also think about all kinds of data. Obviously, they are not the typical kinds of IT data. Here we’re talking about an amazing amount of operational technology, OT data, or in some cases also engineering technology, ET data, things like diagrams, piping diagrams and things like that. So first of all, I think from a data standpoint, I think there’s just an enormous amount of value in these traditional industries, which is, I believe, truly underutilized.
And I think on the robotics and AI front, I definitely see the similar patterns that Julie was describing. I think using robots in multiple different ways on the factory shop floor, I think this is how the different industries are leveraging technology in this kind of underutilized space. For example, using robots in dangerous settings to help humans do these kinds of jobs more effectively. I always talk about one of the clients that we work with in Asia, they’re actually in the business of manufacturing sanitary water. So in that case, glazing is actually the process of applying a glazed slurry on the surface of shaped ceramics. It’s a century-old kind of thing, a technical thing that humans have been doing. But since ancient times, a brush was used and hazardous glazing processes can cause disease in workers.
Now, glazing application robots have taken over. These robots can spray the glaze with three times the efficiency of humans with 100% uniformity rate. It’s just one of the many, many examples on the shop floor in heavy manufacturing. Now robots are taking over what humans used to do. And robots and humans work together to make this safer for humans and at the same time produce better products for consumers. So, this is the kind of exciting thing that I’m seeing how AI brings benefits, tangible benefits to the society, to human beings.
Laurel: That’s a really interesting kind of shift into this next topic, which is how do we then talk about, as you mentioned, being responsible and having ethical AI, especially when we’re discussing making people’s jobs better, safer, more consistent? And then how does this also play into responsible technology in general and how we’re looking at the entire field?
Lan: Yeah, that’s a super hot topic. Okay, I would say as an AI practitioner, responsible AI has always been at the top of the mind for us. But think about the recent advancement in generative AI. I think this topic is becoming even more urgent. So, while technical advancements in AI are very impressive like many examples I’ve been talking about, I think responsible AI is not purely a technical pursuit. It’s also about how we use it, how each of us uses it as a consumer, as a business leader.
So at Accenture, our teams strive to design, build, and deploy AI in a manner that empowers employees and business and fairly impacts customers and society. I think that responsible AI not only applies to us but is also at the core of how we help clients innovate. As they look to scale their use of AI, they want to be confident that their systems are going to perform reliably and as expected. Part of building that confidence, I believe, is ensuring they have taken steps to avoid unintended consequences. That means making sure that there’s no bias in their data and models and that the data science team has the right skills and processes in place to produce more responsible outputs. Plus, we also make sure that there are governance structures for where and how AI is applied, especially when AI systems are using decision-making that affects people’s life. So, there are many, many examples of that.
And I think given the recent excitement around generative AI, this topic becomes even more important, right? What we are seeing in the industry is this is becoming one of the first questions that our clients ask us to help them get generative AI ready. And simply because there are newer risks, newer limitations being introduced because of the generative AI in addition to some of the known or existing limitations in the past when we talk about predictive or prescriptive AI. For example, misinformation. Your AI could, in this case, be producing very accurate results, but if the information generated or content generated by AI is not aligned to human values, is not aligned to your company core values, then I don’t think it’s working, right? It could be a very accurate model, but we also need to pay attention to potential misinformation, misalignment. That’s one example.
Second example is language toxicity. Again, in the traditional or existing AI’s case, when AI is not producing content, language of toxicity is less of an issue. But now this is becoming something that is top of mind for many business leaders, which means responsible AI also needs to cover this new set of a risk, potential limitations to address language toxicity. So those are the couple thoughts I have on the responsible AI.
Laurel: And Julie, you discussed how robots and humans can work together. So how do you think about changing the perception of the fields? How can ethical AI and even governance help researchers and not hinder them with all this great new technology?
Julie: Yeah. I fully agree with Lan’s comments here and have spent quite a fair amount of effort over the past few years on this topic. I recently spent three years as an associate dean at MIT, building out our new cross-disciplinary program and social and ethical responsibilities of computing. This is a program that has involved very deeply, nearly 10% of the faculty researchers at MIT, not just technologists, but social scientists, humanists, those from the business school. And what I’ve taken away is, first of all, there’s no codified process or rule book or design guidance on how to anticipate all of the currently unknown unknowns. There’s no world in which a technologist or an engineer sits on their own or discusses or aims to envision possible futures with those within the same disciplinary background or other sort of homogeneity in background and is able to foresee the implications for other groups and the broader implications of these technologies.
The first question is, what are the right questions to ask? And then the second question is, who has methods and insights to be able to bring to bear on this across disciplines? And that’s what we’ve aimed to pioneer at MIT, is to really bring this sort of embedded approach to drawing in the scholarship and insight from those in other fields in academia and those from outside of academia and bring that into our practice in engineering new technologies.
And just to give you a concrete example of how hard it is to even just determine whether you’re asking the right question, for the technologies that we develop in my lab, we believed for many years that the right question was, how do we develop and shape technologies so that it augments rather than replaces? And that’s been the public discourse about robots and AI taking people’s jobs. “What’s going to happen 10 years from now? What’s happening today?” with well-respected studies put out a few years ago that for every one robot you introduced into a community, that community loses up to six jobs.
So, what I learned through deep engagement with scholars from other disciplines here at MIT as a part of the Work of the Future task force is that that’s actually not the right question. So as it turns out, you just take manufacturing as an example because there’s very good data there. In manufacturing broadly, only one in 10 firms have a single robot, and that’s including the very large firms that make high use of robots like automotive and other fields. And then when you look at small and medium firms, those are 500 or fewer employees, there’s essentially no robots anywhere. And there’s significant challenges in upgrading technology, bringing the latest technologies into these firms. These firms represent 98% of all manufacturers in the US and are coming up on 40% to 50% of the manufacturing workforce in the U.S. There’s good data that the lagging, technological upgrading of these firms is a very serious competitiveness issue for these firms.
And so what I learned through this deep collaboration with colleagues from other disciplines at MIT and elsewhere is that the question isn’t “How do we address the problem we’re creating about robots or AI taking people’s jobs?” but “Are robots and the technologies we’re developing actually doing the job that we need them to do and why are they actually not useful in these settings?”. And you have these really exciting case stories of the few cases where these firms are able to bring in, implement and scale these technologies. They see a whole host of benefits. They don’t lose jobs, they are able to take on more work, they’re able to bring on more workers, those workers have higher wages, the firm is more productive. So how do you realize this sort of win-win-win situation and why is it that so few firms are able to achieve that win-win-win situation?
There’s many different factors. There’s organizational and policy factors, but there are actually technological factors as well that we now are really laser focused on in the lab in aiming to address how you enable those with the domain expertise, but not necessarily engineering or robotics or programming expertise to be able to program the system, program the task rather than program the robot. It’s a humbling experience for me to believe I was asking the right questions and engaging in this research and really understand that the world is a much more nuanced and complex place and we’re able to understand that much better through these collaborations across disciplines. And that comes back to directly shape the work we do and the impact we have on society.
And so we have a really exciting program at MIT training the next generation of engineers to be able to communicate across disciplines in this way and the future generations will be much better off for it than the training those of us engineers have received in the past.
Lan: Yeah, I think Julie you brought such a great point, right? I think it resonated so well with me. I don’t think this is something that you only see in academia’s kind of setting, right? I think this is exactly the kind of change I’m seeing in industry too. I think how the different roles within the artificial intelligence space come together and then work in a highly collaborative kind of way around this kind of amazing technology, this is something that I’ll admit I’d never seen before. I think in the past, AI seemed to be perceived as something that only a small group of deep researchers or deep scientists would be able to do, almost like, “Oh, that’s something that they do in the lab.” I think that’s kind of a lot of the perception from my clients. That’s why in order to scale AI in enterprise settings has been a huge challenge.
I think with the recent advancement in foundational models, large language models, all these pre-trained models that large tech companies have been building, and obviously academic institutions are a huge part of this, I’m seeing more open innovation, a more open collaborative kind of way of working in the enterprise setting too. I love what you described earlier. It’s a multi-disciplinary kind of thing, right? It’s not like AI, you go to computer science, you get an advanced degree, then that’s the only path to do AI. What we are seeing also in business setting is people, leaders with multiple backgrounds, multiple disciplines within the organization come together is computer scientists, is AI engineers, is social scientists or even behavioral scientists who are really, really good at defining different kinds of experimentation to play with this kind of AI in early-stage statisticians. Because at the end of the day, it’s about probability theory, economists, and of course also engineers.
So even within a company setting in the industries, we are seeing a more open kind of attitude for everyone to come together to be around this kind of amazing technology to all contribute. We always talk about a hub and spoke model. I actually think that this is happening, and everybody is getting excited about technology, rolling up their sleeves and bringing their different backgrounds and skill sets to all contribute to this. And I think this is a critical change, a culture shift that we have seen in the business setting. That’s why I am so optimistic about this positive sum game that we talked about earlier, which is the ultimate impact of the technology.
Laurel: That’s a really great point. Julie, Lan mentioned it earlier, but also this access for everyone to some of these technologies like generative AI and AI chatbots can help everyone build new ideas and explore and experiment. But how does it really help researchers build and adopt those kinds of emerging AI technologies that everyone’s keeping a close eye on the horizon?
Julie: Yeah. Yeah. So, talking about generative AI, for the past 10 or 15 years, every single year I thought I was working in the most exciting time possible in this field. And then it just happens again. For me the really interesting aspect, or one of the really interesting aspects, of generative AI and GPT and ChatGPT is, one, as you mentioned, it’s really in the hands of the public to be able to interact with it and envision multitude of ways it could potentially be useful. But from the work we’ve been doing in what we call positive-sum automation, that’s around these sectors where performance matters a lot, reliability matters a lot. You think about manufacturing, you think about aerospace, you think about healthcare. The introduction of automation, AI, robotics has indexed on that and at the cost of flexibility. And so a part of our research agenda is aiming to achieve the best of both those worlds.
The generative capability is very interesting to me because it’s another point in this space of high performance versus flexibility. This is a capability that is very, very flexible. That’s the idea of training these foundation models and everybody can get a direct sense of that from interacting with it and playing with it. This is not a scenario anymore where we’re very carefully crafting the system to perform at very high capability on very, very specific tasks. It’s very flexible in the tasks you can envision making use of it for. And that’s game changing for AI, but on the flip side of that, the failure modes of the system are very difficult to predict.
So, for high stakes applications, you’re never really developing the capability of doing some specific task in isolation. You’re thinking from a systems perspective and how you bring the relative strengths and weaknesses of different components together for overall performance. The way you need to architect this capability within a system is very different than other forms of AI or robotics or automation because you have a capability that’s very flexible now, but also unpredictable in how it will perform. And so you need to design the rest of the system around that, or you need to carve out the aspects or tasks where failure in particular modes are not critical.
So chatbots for example, by and large, for many of their uses, they can be very helpful in driving engagement and that’s of great benefit for some products or some organizations. But being able to layer in this technology with other AI technologies that don’t have these particular failure modes and layer them in with human oversight and supervision and engagement becomes really important. So how you architect the overall system with this new technology, with these very different characteristics I think is very exciting and very new. And even on the research side, we’re just scratching the surface on how to do that. There’s a lot of room for a study of best practices here particularly in these more high stakes application areas.
Lan: I think Julie makes such a great point that’s super resonating with me. I think, again, always I’m just seeing the exact same thing. I love the couple keywords that she was using, flexibility, positive-sum automation. I think there are two colors I want to add there. I think on the flexibility frame, I think this is exactly what we are seeing. Flexibility through specialization, right? Used with the power of generative AI. I think another term that came to my mind is this resilience, okay? So now AI becomes more specialized, right? AI and humans actually become more specialized. And so that we can both focus on things, little skills or roles, that we’re the best at.
In Accenture, we just recently published our point of view, “A new era of generative AI for everybody.” Within the point of view, we laid out this, what I call the ACCAP framework. It basically addresses, I think, similar points that Julie was talking about. So basically advice, create, code, and then automate, and then protect. If you link all these five, the first letter of these five words together is what I call the ACCAP framework (so that I can remember those five things). But I think this is how different ways we are seeing how AI and humans working together manifest this kind of collaboration in different ways.
For example, advising, it’s pretty obvious with generative AI capabilities. I think the chatbot example that Julie was talking about earlier. Now imagine every role, every knowledge worker’s role in an organization will have this co-pilot, running behind the scenes. In a contact center’s case it could be, okay, now you’re getting this generative AI doing auto summarization of the agent calls with customers at the end of the calls. So the agent doesn’t have to be spending time and doing this manually. And then customers will get happier because customer sentiment will get better detected by generative AI, creating obviously the numerous, even consumer-centric kind of cases around how human creativity is getting unleashed.
And there’s also business examples in marketing, in hyper-personalization, how this kind of creativity by AI is being best utilized. I think automating—again, we’ve been talking about robotics, right? So again, how robots and humans work together to take over some of these mundane tasks. But even in generative AI’s case is not even just the blue-collar kind of jobs, more mundane tasks, also looking into more mundane routine tasks in knowledge worker spaces. I think those are the couple examples that I have in mind when I think of the word flexibility through specialization.
And by doing so, new roles are going to get created. From our perspective, we’ve been focusing on prompt engineering as a new discipline within the AI space—AI ethics specialist. We also believe that this role is going to take off very quickly simply because of the responsible AI topics that we just talked about.
And also because all this business processes have become more efficient, more optimized, we believe that new demand, not just the new roles, each company, regardless of what industries you are in, if you become very good at mastering, harnessing the power of this kind of AI, the new demand is going to create it. Because now your products are getting better, you are able to provide a better experience to your customer, your pricing is going to get optimized. So I think bringing this together is, which is my second point, this will bring positive sum to the society in economics kind of terms where we’re talking about this. Now you’re pushing out the production possibility frontier for the society as a whole.
So, I’m very optimistic about all these amazing aspects of flexibility, resilience, specialization, and also generating more economic profit, economic growth for the society aspect of AI. As long as we walk into this with eyes wide open so that we understand some of the existing limitations, I’m sure we can do both of them.
Laurel: And Julie, Lan just laid out this fantastic, really a correlation of generative AI as well as what’s possible in the future. What are you thinking about artificial intelligence and the opportunities in the next three to five years?
Julie: Yeah. Yeah. So, I think Lan and I are very largely on the same page on just about all of these topics, which is really great to hear from the academic and the industry side. Sometimes it can feel as though the emergence of these technologies is just going to sort of steamroll and work and jobs are going to change in some predetermined way because the technology now exists. But we know from the research that the data doesn’t bear that out actually. There’s many, many decisions you make in how you design, implement, and deploy, and even make the business case for these technologies that can really sort of change the course of what you see in the world because of them. And for me, I really think a lot about this question of what’s called lights out in manufacturing, like lights out operation where there’s this idea that with the advances and all these capabilities, you would aim to be able to run everything without people at all. So, you don’t need lights on for the people.
And again, as a part of the Work of the Future task force and the research that we’ve done visiting companies, manufacturers, OEMs, suppliers, large international or multinational firms as well as small and medium firms across the world, the research team asked this question of, “So these high performers that are adopting new technologies and doing well with it, where is all this headed? Is this headed towards a lights out factory for you?” And there were a variety of answers. So some people did say, “Yes, we’re aiming for a lights out factory,” but actually many said no, that that was not the end goal. And one of the quotes, one of the interviewees stopped while giving a tour and turned around and said, “A lights out factory. Why would I want a lights out factory? A factory without people is a factory that’s not innovating.”
I think that’s the core for me, the core point of this. When we deploy robots, are we caging and sort of locking the people out of that process? When we deploy AI, is essentially the infrastructure and data curation process so intensive that it really locks out the ability for a domain expert to come in and understand the process and be able to engage and innovate? And so for me, I think the most exciting research directions are the ones that enable us to pursue this sort of human-centered approach to adoption and deployment of the technology and that enable people to drive this innovation process. So a factory, there’s a well-defined productivity curve. You don’t get your assembly process when you start. That’s true in any job or any field. You never get it exactly right or you optimize it to start, but it’s a very human process to improve. And how do we develop these technologies such that we’re maximally leveraging our human capability to innovate and improve how we do our work?
My view is that by and large, the technologies we have today are really not designed to support that and they really impede that process in a number of different ways. But you do see increasing investment and exciting capabilities in which you can engage people in this human-centered process and see all the benefits from that. And so for me, on the technology side and shaping and developing new technologies, I’m most excited about the technologies that enable that capability.
Laurel: Excellent. Julie and Lan, thank you so much for joining us today on what’s been a really fantastic episode of The Business Lab.
Julie: Thank you so much for having us.
Lan: Thank you.
Laurel: That was Lan Guan of Accenture and Julie Shah of MIT who I spoke with from Cambridge, Massachusetts, the home of MIT and MIT Technology Review overlooking the Charles River.
That’s it for this episode of Business Lab. I’m your host, Laurel Ruma. I’m the director of Insights, the custom publishing division of MIT Technology Review. We were founded in 1899 at the Massachusetts Institute of Technology. You can find us in print, on the web, and at events each year around the world. For more information about us and the show, please check out our website at technologyreview.com.
This show is available wherever you get your podcasts. If you enjoyed this episode, we hope you’ll take a moment to rate and review us. Business Lab is a production of MIT Technology Review. This episode was produced by Giro Studios. Thanks for listening.
This content was produced by Insights, the custom content arm of MIT Technology Review. It was not written by MIT Technology Review’s editorial staff.
You may like
-
Axis bank delights customers with a cloud-first approach to digital transformation
-
Long COVID: Scientists Develop New Approach To Better Understand Mysterious Condition
-
More Rapid COVID-19 Testing Approach Detects Infection Hours After Exposure
-
How to Use the 80/20 Approach to Become a Better Runner
-
Adopting public cloud for automotive manufacturing operations
-
Responsible adoption of AI: A cloud-centric approach
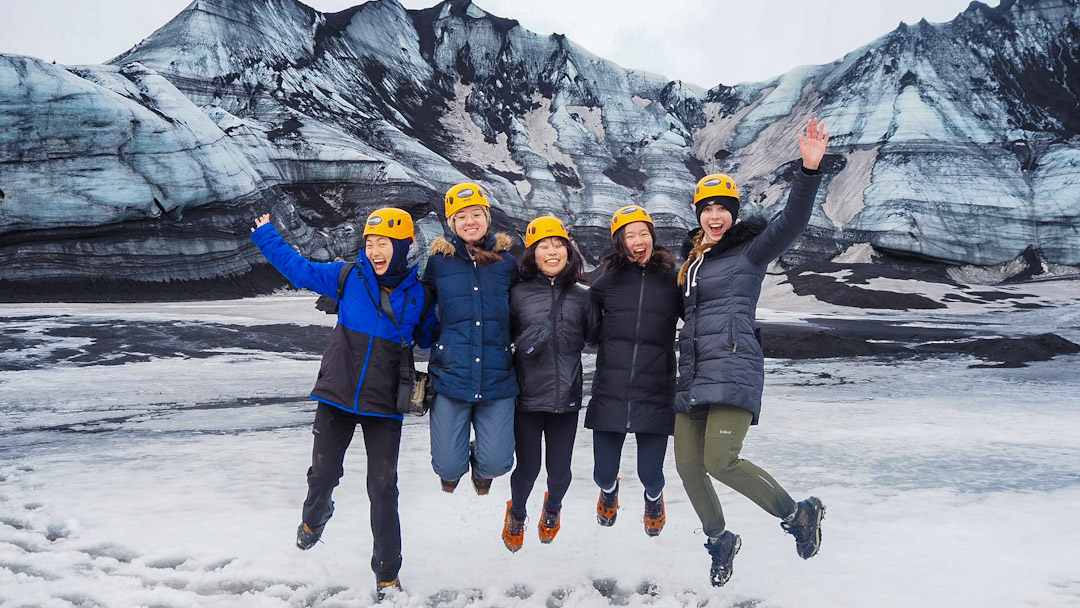
My senior spring in high school, I decided to defer my MIT enrollment by a year. I had always planned to take a gap year, but after receiving the silver tube in the mail and seeing all my college-bound friends plan out their classes and dorm decor, I got cold feet. Every time I mentioned my plans, I was met with questions like “But what about school?” and “MIT is cool with this?”
Yeah. MIT totally is. Postponing your MIT start date is as simple as clicking a checkbox.
COURTESY PHOTO
Now, having finished my first year of classes, I’m really grateful that I stuck with my decision to delay MIT, as I realized that having a full year of unstructured time is a gift. I could let my creative juices run. Pick up hobbies for fun. Do cool things like work at an AI startup and teach myself how to create latte art. My favorite part of the year, however, was backpacking across Europe. I traveled through Austria, Slovakia, Russia, Spain, France, the UK, Greece, Italy, Germany, Poland, Romania, and Hungary.
Moreover, despite my fear that I’d be losing a valuable year, traveling turned out to be the most productive thing I could have done with my time. I got to explore different cultures, meet new people from all over the world, and gain unique perspectives that I couldn’t have gotten otherwise. My travels throughout Europe allowed me to leave my comfort zone and expand my understanding of the greater human experience.
“In Iceland there’s less focus on hustle culture, and this relaxed approach to work-life balance ends up fostering creativity. This was a wild revelation to a bunch of MIT students.”
When I became a full-time student last fall, I realized that StartLabs, the premier undergraduate entrepreneurship club on campus, gives MIT undergrads a similar opportunity to expand their horizons and experience new things. I immediately signed up. At StartLabs, we host fireside chats and ideathons throughout the year. But our flagship event is our annual TechTrek over spring break. In previous years, StartLabs has gone on TechTrek trips to Germany, Switzerland, and Israel. On these fully funded trips, StartLabs members have visited and collaborated with industry leaders, incubators, startups, and academic institutions. They take these treks both to connect with the global startup sphere and to build closer relationships within the club itself.
Most important, however, the process of organizing the TechTrek is itself an expedited introduction to entrepreneurship. The trip is entirely planned by StartLabs members; we figure out travel logistics, find sponsors, and then discover ways to optimize our funding.
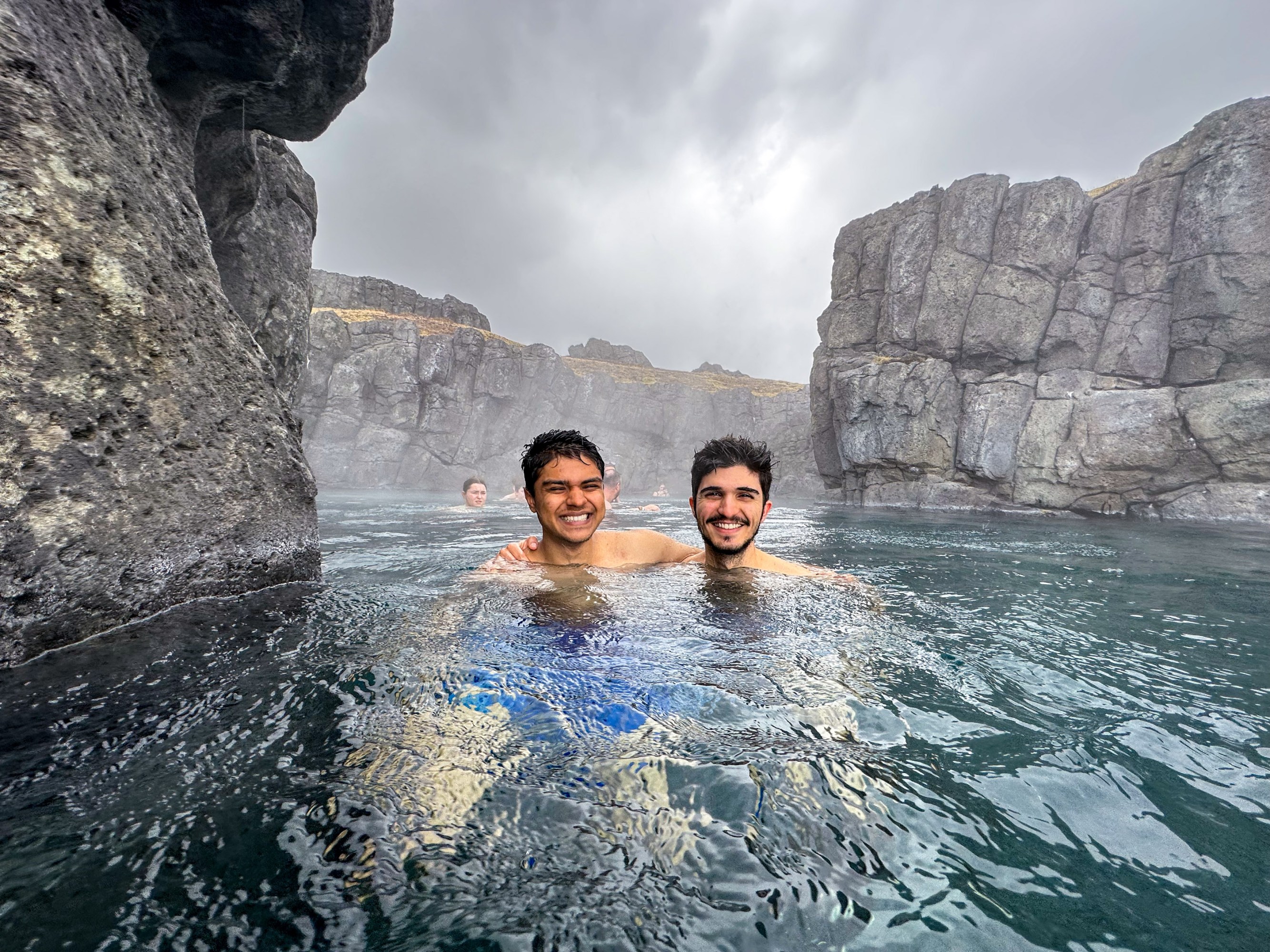
COURTESY PHOTO
In organizing this year’s trip to Iceland, we had to learn how to delegate roles to all the planners and how to maintain morale when making this trip a reality seemed to be an impossible task. We woke up extra early to take 6 a.m. calls with Icelandic founders and sponsors. We came up with options for different levels of sponsorship, used pattern recognition to deduce the email addresses of hundreds of potential contacts at organizations we wanted to visit, and all got scrappy with utilizing our LinkedIn connections.
And as any good entrepreneur must, we had to learn how to be lean and maximize our resources. To stretch our food budget, we planned all our incubator and company visits around lunchtime in hopes of getting fed, played human Tetris as we fit 16 people into a six-person Airbnb, and emailed grocery stores to get their nearly expired foods for a discount. We even made a deal with the local bus company to give us free tickets in exchange for a story post on our Instagram account.
Tech
The Download: spying keyboard software, and why boring AI is best
Published
8 months agoon
22 August 2023By
Terry Power
This is today’s edition of The Download, our weekday newsletter that provides a daily dose of what’s going on in the world of technology.
How ubiquitous keyboard software puts hundreds of millions of Chinese users at risk
For millions of Chinese people, the first software they download onto devices is always the same: a keyboard app. Yet few of them are aware that it may make everything they type vulnerable to spying eyes.
QWERTY keyboards are inefficient as many Chinese characters share the same latinized spelling. As a result, many switch to smart, localized keyboard apps to save time and frustration. Today, over 800 million Chinese people use third-party keyboard apps on their PCs, laptops, and mobile phones.
But a recent report by the Citizen Lab, a University of Toronto–affiliated research group, revealed that Sogou, one of the most popular Chinese keyboard apps, had a massive security loophole. Read the full story.
—Zeyi Yang
Why we should all be rooting for boring AI
Earlier this month, the US Department of Defense announced it is setting up a Generative AI Task Force, aimed at “analyzing and integrating” AI tools such as large language models across the department. It hopes they could improve intelligence and operational planning.
But those might not be the right use cases, writes our senior AI reporter Melissa Heikkila. Generative AI tools, such as language models, are glitchy and unpredictable, and they make things up. They also have massive security vulnerabilities, privacy problems, and deeply ingrained biases.
Applying these technologies in high-stakes settings could lead to deadly accidents where it’s unclear who or what should be held responsible, or even why the problem occurred. The DoD’s best bet is to apply generative AI to more mundane things like Excel, email, or word processing. Read the full story.
This story is from The Algorithm, Melissa’s weekly newsletter giving you the inside track on all things AI. Sign up to receive it in your inbox every Monday.
The ice cores that will let us look 1.5 million years into the past
To better understand the role atmospheric carbon dioxide plays in Earth’s climate cycles, scientists have long turned to ice cores drilled in Antarctica, where snow layers accumulate and compact over hundreds of thousands of years, trapping samples of ancient air in a lattice of bubbles that serve as tiny time capsules.
By analyzing those cores, scientists can connect greenhouse-gas concentrations with temperatures going back 800,000 years. Now, a new European-led initiative hopes to eventually retrieve the oldest core yet, dating back 1.5 million years. But that impressive feat is still only the first step. Once they’ve done that, they’ll have to figure out how they’re going to extract the air from the ice. Read the full story.
—Christian Elliott
This story is from the latest edition of our print magazine, set to go live tomorrow. Subscribe today for as low as $8/month to ensure you receive full access to the new Ethics issue and in-depth stories on experimental drugs, AI assisted warfare, microfinance, and more.
The must-reads
I’ve combed the internet to find you today’s most fun/important/scary/fascinating stories about technology.
1 How AI got dragged into the culture wars
Fears about ‘woke’ AI fundamentally misunderstand how it works. Yet they’re gaining traction. (The Guardian)
+ Why it’s impossible to build an unbiased AI language model. (MIT Technology Review)
2 Researchers are racing to understand a new coronavirus variant
It’s unlikely to be cause for concern, but it shows this virus still has plenty of tricks up its sleeve. (Nature)
+ Covid hasn’t entirely gone away—here’s where we stand. (MIT Technology Review)
+ Why we can’t afford to stop monitoring it. (Ars Technica)
3 How Hilary became such a monster storm
Much of it is down to unusually hot sea surface temperatures. (Wired $)
+ The era of simultaneous climate disasters is here to stay. (Axios)
+ People are donning cooling vests so they can work through the heat. (Wired $)
4 Brain privacy is set to become important
Scientists are getting better at decoding our brain data. It’s surely only a matter of time before others want a peek. (The Atlantic $)
+ How your brain data could be used against you. (MIT Technology Review)
5 How Nvidia built such a big competitive advantage in AI chips
Today it accounts for 70% of all AI chip sales—and an even greater share for training generative models. (NYT $)
+ The chips it’s selling to China are less effective due to US export controls. (Ars Technica)
+ These simple design rules could turn the chip industry on its head. (MIT Technology Review)
6 Inside the complex world of dissociative identity disorder on TikTok
Reducing stigma is great, but doctors fear people are self-diagnosing or even imitating the disorder. (The Verge)
7 What TikTok might have to give up to keep operating in the US
This shows just how hollow the authorities’ purported data-collection concerns really are. (Forbes)
8 Soldiers in Ukraine are playing World of Tanks on their phones
It’s eerily similar to the war they are themselves fighting, but they say it helps them to dissociate from the horror. (NYT $)
9 Conspiracy theorists are sharing mad ideas on what causes wildfires
But it’s all just a convoluted way to try to avoid having to tackle climate change. (Slate $)
10 Christie’s accidentally leaked the location of tons of valuable art
Seemingly thanks to the metadata that often automatically attaches to smartphone photos. (WP $)
Quote of the day
“Is it going to take people dying for something to move forward?”
—An anonymous air traffic controller warns that staffing shortages in their industry, plus other factors, are starting to threaten passenger safety, the New York Times reports.
The big story
Inside effective altruism, where the far future counts a lot more than the present

October 2022
Since its birth in the late 2000s, effective altruism has aimed to answer the question “How can those with means have the most impact on the world in a quantifiable way?”—and supplied methods for calculating the answer.
It’s no surprise that effective altruisms’ ideas have long faced criticism for reflecting white Western saviorism, alongside an avoidance of structural problems in favor of abstract math. And as believers pour even greater amounts of money into the movement’s increasingly sci-fi ideals, such charges are only intensifying. Read the full story.
—Rebecca Ackermann
We can still have nice things
A place for comfort, fun and distraction in these weird times. (Got any ideas? Drop me a line or tweet ’em at me.)
+ Watch Andrew Scott’s electrifying reading of the 1965 commencement address ‘Choose One of Five’ by Edith Sampson.
+ Here’s how Metallica makes sure its live performances ROCK. ($)
+ Cannot deal with this utterly ludicrous wooden vehicle.
+ Learn about a weird and wonderful new instrument called a harpejji.
Tech
Why we should all be rooting for boring AI
Published
8 months agoon
22 August 2023By
Terry Power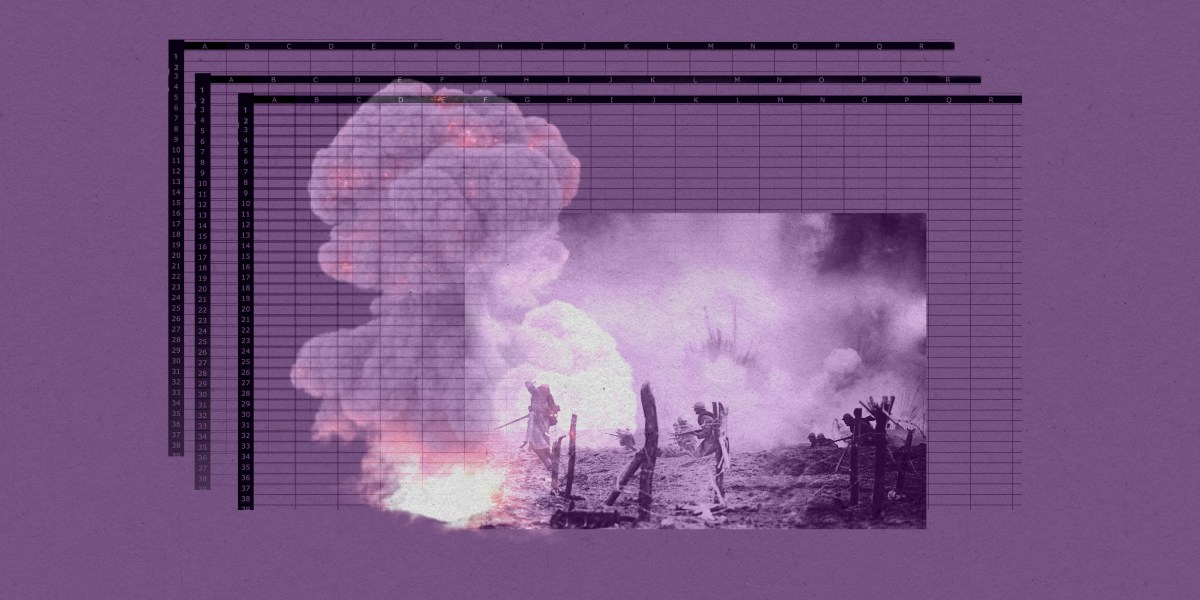
This story originally appeared in The Algorithm, our weekly newsletter on AI. To get stories like this in your inbox first, sign up here.
I’m back from a wholesome week off picking blueberries in a forest. So this story we published last week about the messy ethics of AI in warfare is just the antidote, bringing my blood pressure right back up again.
Arthur Holland Michel does a great job looking at the complicated and nuanced ethical questions around warfare and the military’s increasing use of artificial-intelligence tools. There are myriad ways AI could fail catastrophically or be abused in conflict situations, and there don’t seem to be any real rules constraining it yet. Holland Michel’s story illustrates how little there is to hold people accountable when things go wrong.
Last year I wrote about how the war in Ukraine kick-started a new boom in business for defense AI startups. The latest hype cycle has only added to that, as companies—and now the military too—race to embed generative AI in products and services.
Earlier this month, the US Department of Defense announced it is setting up a Generative AI Task Force, aimed at “analyzing and integrating” AI tools such as large language models across the department.
The department sees tons of potential to “improve intelligence, operational planning, and administrative and business processes.”
But Holland Michel’s story highlights why the first two use cases might be a bad idea. Generative AI tools, such as language models, are glitchy and unpredictable, and they make things up. They also have massive security vulnerabilities, privacy problems, and deeply ingrained biases.
Applying these technologies in high-stakes settings could lead to deadly accidents where it’s unclear who or what should be held responsible, or even why the problem occurred. Everyone agrees that humans should make the final call, but that is made harder by technology that acts unpredictably, especially in fast-moving conflict situations.
Some worry that the people lowest on the hierarchy will pay the highest price when things go wrong: “In the event of an accident—regardless of whether the human was wrong, the computer was wrong, or they were wrong together—the person who made the ‘decision’ will absorb the blame and protect everyone else along the chain of command from the full impact of accountability,” Holland Michel writes.
The only ones who seem likely to face no consequences when AI fails in war are the companies supplying the technology.
It helps companies when the rules the US has set to govern AI in warfare are mere recommendations, not laws. That makes it really hard to hold anyone accountable. Even the AI Act, the EU’s sweeping upcoming regulation for high-risk AI systems, exempts military uses, which arguably are the highest-risk applications of them all.
While everyone is looking for exciting new uses for generative AI, I personally can’t wait for it to become boring.
Amid early signs that people are starting to lose interest in the technology, companies might find that these sorts of tools are better suited for mundane, low-risk applications than solving humanity’s biggest problems.
Applying AI in, for example, productivity software such as Excel, email, or word processing might not be the sexiest idea, but compared to warfare it’s a relatively low-stakes application, and simple enough to have the potential to actually work as advertised. It could help us do the tedious bits of our jobs faster and better.
Boring AI is unlikely to break as easily and, most important, won’t kill anyone. Hopefully, soon we’ll forget we’re interacting with AI at all. (It wasn’t that long ago when machine translation was an exciting new thing in AI. Now most people don’t even think about its role in powering Google Translate.)
That’s why I’m more confident that organizations like the DoD will find success applying generative AI in administrative and business processes.
Boring AI is not morally complex. It’s not magic. But it works.
Deeper Learning
AI isn’t great at decoding human emotions. So why are regulators targeting the tech?
Amid all the chatter about ChatGPT, artificial general intelligence, and the prospect of robots taking people’s jobs, regulators in the EU and the US have been ramping up warnings against AI and emotion recognition. Emotion recognition is the attempt to identify a person’s feelings or state of mind using AI analysis of video, facial images, or audio recordings.
But why is this a top concern? Western regulators are particularly concerned about China’s use of the technology, and its potential to enable social control. And there’s also evidence that it simply does not work properly. Tate Ryan-Mosley dissected the thorny questions around the technology in last week’s edition of The Technocrat, our weekly newsletter on tech policy.
Bits and Bytes
Meta is preparing to launch free code-generating software
A version of its new LLaMA 2 language model that is able to generate programming code will pose a stiff challenge to similar proprietary code-generating programs from rivals such as OpenAI, Microsoft, and Google. The open-source program is called Code Llama, and its launch is imminent, according to The Information. (The Information)
OpenAI is testing GPT-4 for content moderation
Using the language model to moderate online content could really help alleviate the mental toll content moderation takes on humans. OpenAI says it’s seen some promising first results, although the tech does not outperform highly trained humans. A lot of big, open questions remain, such as whether the tool can be attuned to different cultures and pick up context and nuance. (OpenAI)
Google is working on an AI assistant that offers life advice
The generative AI tools could function as a life coach, offering up ideas, planning instructions, and tutoring tips. (The New York Times)
Two tech luminaries have quit their jobs to build AI systems inspired by bees
Sakana, a new AI research lab, draws inspiration from the animal kingdom. Founded by two prominent industry researchers and former Googlers, the company plans to make multiple smaller AI models that work together, the idea being that a “swarm” of programs could be as powerful as a single large AI model. (Bloomberg)