Tech
Engineering the future of mobility
Published
2 years agoon
By
Terry Power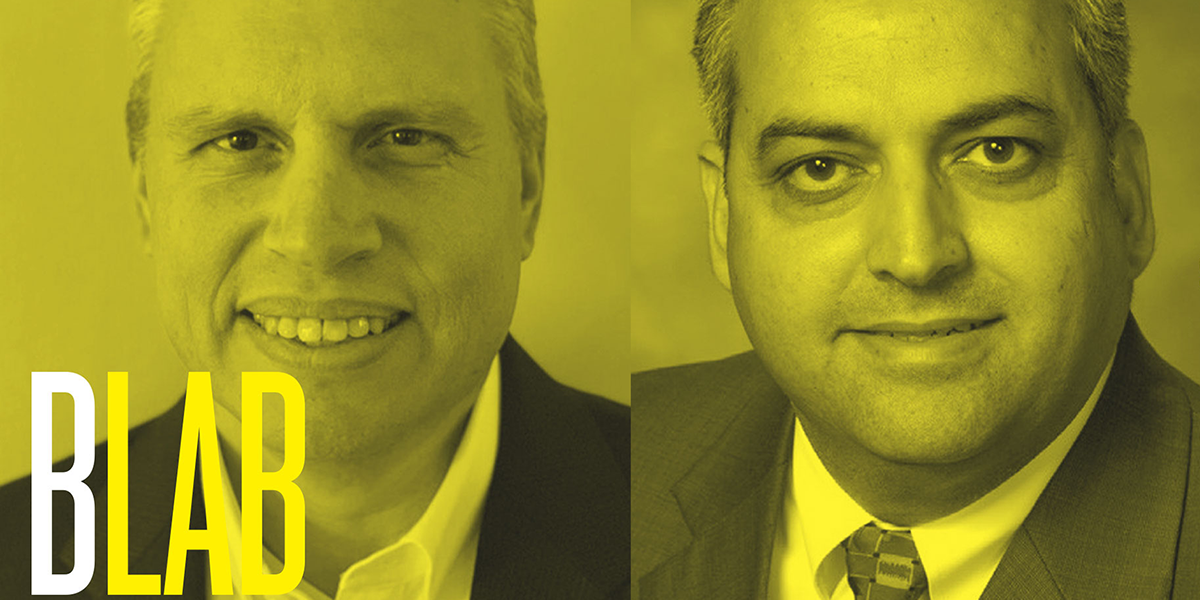
From cars to planes, the future of transportation is already here—and is changing rapidly. Software engineering is increasingly central to both the development and maintenance of all kinds of vehicles. That means more people need to start thinking like systems engineers. Dale Tutt, vice president of aerospace and defense industry for Siemens Software, says this means companies must offer more training and planning for those designing and developing vehicles of the future.
“As you try to address the talent gap, there’s a lot you can do to help make the tools easier to use. By better integrating the tools and by bringing in technologies like AI to help automate the generation of different design concepts and the analysis of those concepts using simulation tools, you can extend the capabilities of the system so that it helps empower your engineers,” says Tutt.
“Companies that are the most successful at adopting systems engineering are doing it because systems engineering, and the tools being used are becoming almost like the DNA of their engineering organization. Everyone is starting to think a bit like a systems engineer, even in their normal job. The tools and the ecosystem that you use to do systems engineering has a large role in facilitating adoption.”
Nand Kochhar, the vice president of automotive and transportation for Siemens Software, says a systems engineering approach can extend more broadly, as engineers think about how cars and vehicles connect to everything else in their environments.
“In a smart city, the system has become the city itself. Take a vehicle in the city, for example. The definition of the system has moved from the single vehicle to include the flow of traffic in the city and to how the traffic lights operate. You can extend that expansive ecosystem to other aspects like building management, for example, into the smart city environment,” he says.
“It becomes a totally different business case than what we have today. These new technologies are furthering innovation, both at the technical level as well as at a business model level. So, as a result of autonomy and the autonomous vehicle deployment, new business models are being formed.”
Full transcript
Laurel Ruma: From MIT Technology Review. I’m Laurel Ruma, and this is Business Lab, the show that helps business leaders make sense of new technologies coming out of the lab and into the marketplace.
Our topic today is the software-driven engineering environment. How a car or a plane is built now is much different than in the days of Henry Ford and the Wright Brothers. Vehicles and planes now have more software than hardware. As innovation evolves, the complexity of the software evolves as well, which allows for new types of inventions.
Two words for you: systems engineering.
My guests today are Nand Kochhar and Dale Tutt. Nand is the vice president of automotive and transportation for Siemens Software. He joined Siemens in 2020 after almost 30 years at Ford Motor Company, where he held a number of positions, including global safety systems chief engineer, and executive technical lead.
Dale Tutt is the vice president of aerospace and defense industry for Siemens Software. Prior to this role, Dale worked at The Spaceship Company. And in December 2018, he led the team on a successful flight to space. Welcome Nand and Dale.
Nand Kochhar: Thank you, Laurel.
Dale Tutt: Thank you, Laurel, we’re very excited to be here today.
Laurel: So, as product development across industries—including aerospace and defense, and automotive—transitions from mechanical engineering to a software-driven engineering environment, systems are growing in complexity. Software-driven engineering has given rise to the interdisciplinary field of systems engineering. In what way has this new field or new approach affected the industry as a whole—and aerospace and defense, and automotive, in particular?
Nand: This field has provided an opportunity for the automotive industry to continue to innovate. As you know, the trends in the industry are changing—we’re moving from internal combustion engines to new propulsion systems, going toward electrification. Our vehicles are also adapting to levels of autonomy, from SAE Level 1 to 5.
All that brings a lot of complexity. In fact, you could say software is eating the car, as software is becoming a predominant part of today’s automobile and future automobile development. The software engineering-based discipline has allowed us to address that growing complexity, and it has allowed our engineers to continue to innovate and offer products the end customer wants. That is the big change—how the product development and manufacturing in the automobile industry is changing.
Dale: As Nand was saying about the automotive industry, we’re seeing a need to innovate much more than we have in the past within aerospace and defense. Whether it’s building new air taxis or the EVTOL aircraft, the advances in space systems and putting more and more people into we’re changing the way we’re doing space exploration.
As we move into the future, the need for more sustainability and to address using less fuel and having greater efficiency in our systems has driven a lot innovation in the industry. Companies are using software to enable much more complex systems with the expectation that they’ll operate more efficiently, more effectively. In the case of aircraft, the expected result is a lower weight aircraft, which then uses less energy to go from one place to the next.
Systems engineering has been predominant in aerospace and defense for decades now. It’s now being used to help drive some of that innovation, to help make sure that we have looked at all of the possible combinations of a complex system, and possible failure modes of those systems, so that we can provide the safest, most reliable, highest-performing product possible for customers. So, while it’s been around for a long time, in looking at these complex systems, we’ve seen a tremendous growth in the need for systems engineering and model-based systems engineering among all of our customers to get the innovation they want to have.
Laurel: So, cars are evolving as are planes, and one could even say that there’s a massive shift, not just from combustion engines to electric vehicles, but also to autonomous vehicles for both of these great innovations. Nand, how does that affect cars in general and when we think about how systems are being used and changing so drastically?
Nand: Yes, as you said, it changes the entire product development approach when you look at autonomous vehicles or electric vehicles. So, let’s take one at a time. In electric vehicles, instead of having the combustion engines, of course, now, there is a battery system as the source of power generation. Then, you have the transmission of that power going through the wheels. So, a lot of the mechanisms in between are changing.
When we say it’s battery powered, to deliver electrification, it’s not just the battery. It is the entire electronics, which changes with it the whole architecture as well as the software. The software is performing what we call battery management because it’s continuously optimizing the operations of the battery so that it can deliver the power on demand and be the most efficient, while still addressing the cross-attribute issues of any thermal performance.
The fundamental shift is happening at a vehicle-attribute level, at a driver-vehicle performance level, and not only at the component level that you now have in rotors and motors and batteries, versus the previous systems. When you look at autonomy, it becomes even more complex, starting with the levels of autonomy to SAE Level 2, where you have both the braking functions as well as the steering functions and the decision-making happening. So, now you have an extra set of sensors in the car. They’re collecting information, collecting data all the time. That information is being sent to a central processing unit to make decisions, so you’ve got an extra set of software, the algorithms, making those decisions. Those decisions are going back into operations, whether it’s braking or whether it’s steering. So, the level of complexity has increased.
You can take it even further to the SAE Level 3 or 4. Now, you have the camera and LIDAR radar. Sensing systems also need to be talking to the infrastructure, whether that is the city traffic lighting or whether it is other parts of the transponders installed within the cities. The definition of the systems have changed. In the past, when we didn’t have advanced vehicles with levels of autonomy, the vehicle itself was called the system. It was a system of systems, and those subsystems were body, chassis, powertrain, electronics. Now, when you look at this autonomous environment, the vehicle itself has become a subsystem, and it is working in the system with other cars on the road and with the infrastructure, so the definition of systems have changed. This is how the approach of system of systems is the only way to address the kind of autonomy we want to enjoy down the road.
Laurel: Dale, with EVTOL, or electric vertical takeoff and landing aircrafts, just one example, is it similar in how the systems of systems are changing and evolving?
Dale: Absolutely. As Nand said, there was, for many years, a lot of focus on the aircraft or the product itself and thinking of it as a system of systems. With the use of drones, that became more of a system of systems problem, and the EVTOLs are a problem that’s very similar to what we’re seeing in the automotive industry when we talk about autonomous vehicles. How do the aircraft interact with the environment with sensors in a city, because you’re going to be flying amongst buildings? You have to be able to sense and avoid other aircraft that are flying. You have to interact with the charging stations that are part of the infrastructure.
It does become a much broader, more complex problem. You have a higher level of connectivity between the different vehicles that are flying around. Then, you need capabilities as simple as being able to track them and enable them to interact with an app on a phone, as people envision something like ridesharing. That is part of how the whole system of systems works. It’s a much more complex problem than we’ve had in the past, and you have to be able to connect all the pieces together and manage them and have them interact properly so that you get the desired performance and usability of the service.
Laurel: Speaking of those types of challenges, as company infrastructures become more like systems of systems to incorporate technologies like AI, artificial intelligence and machine learning, it makes sense perhaps to shift thinking to a systems engineering approach applied to an entire company. What types of technological changes do companies face in integrating systems engineering into an existing architecture?
Dale: There’s always a bit of a cultural challenge, too, as you start to bring in the new systems approach that, sometimes, people just want to jump right in and start designing something. As an engineer, I guess I’ve been guilty of that a few times myself, but you really need to have the systems that help manage your requirements. You can automate the checking of those requirements so that they’re written properly and that they’re decomposing from a system of systems to a product, to the individual subsystems within an aircraft or within a vehicle. So, there is a lot of technology. You have greater interactions between your simulations, the design software that you’re using, and then the tools you’re using to manage the system modeling, but with the higher amount of autonomy that they’re desiring, you start to have more of a system safety influence as well. So, you really need to be able to connect these solutions together so you don’t miss things, so that you can see a complete picture. It’s a lot easier to optimize your products when your software solutions are connected together in a single ecosystem.
That’s the technical side. I mentioned a little bit about the culture and the need for people to change their mindset, to adopt a systems engineering mindset. They no longer are working just on their little piece of the vehicle, but they’re thinking about it in the context of how it influences and interacts with all the other systems on the aircraft—or within the ecosystem, in the case of something like air taxis. You have to look at your processes, you have to look at your people, and you have to look at the technology you’re bringing on board to put together a complete process that empowers the engineers to be more innovative and to think of new solutions.
Laurel: Speaking of that, Nand, what does empowering engineers look like when you’re working on a project?
Nand: From a systems engineering standpoint, allowing them to first define the problem which needs to be solved, then enabling them with the tools and processes required to deliver that, is where empowerment comes into play. There are several levels of technical challenges and solutions, and empowering means enabling them with all those.
Depending on where an individual company or organization is at in its digital transformation journey, those challenges and solutions would be different. From a pure infrastructure or hardware perspective, some will have sufficient hardware installed, which can handle the extensive amount of modeling and computing in their environment. Others will face challenges of making sure there are no silos, to Dale’s point of culture, within the company, and ensuring the information is flowing seamlessly from one end to the other in a digital thread format. Those are the challenges. That’s where it is very important to have an overall plan to have the technology part, as well as the culture and the people side of the business addressed from a talent perspective, in order to deliver a systems engineering approach.
Laurel: A bit more on that, Dale—how do companies look at that kind of challenge, of overcoming the talent gaps and breaking down those information silos? Those are two primary focuses, you could say, of digital transformation across any industry, but specifically for aerospace and defense and automotive. So, it’s a real shift to think of this in a different way.
Dale: Yes. When you start looking at how to address this, you have to go through some amount of training with your people and get them not just to learn the skills, but to adopt the mindset that it takes to be a systems engineer. The other piece of it is looking for the solutions that actually help automate some of those processes.
Sometimes, when you start to break down the barriers, if you think about traditional structural design and structures analysis, like when you’re designing a composite skin panel on an airplane, in the past, the designer would design it in CAD and then hand it off to an analyst to do the stress analysis on the part. Then, they would have to talk back and forth. Now, as you start to bring the tools together and you start to bring the simulation and the design together, you’re now able to start having the same person do both tasks because the tools are easy to use, they’re integrated, and they’re well automated together.
As you extend that into systems engineering, as you try to address the talent gap, there’s only so much you can do with training, but there’s a lot that you can do to help make the tools simpler and easier to use. By making them better integrated, by bringing technologies like AI into it, where you can help automate the generation of different design concepts and the analysis of those concepts using simulation tools, you can extend the capabilities of the system so that it helps empower your engineers.
The companies that are the most successful at adopting systems engineering are doing it because systems engineering and the tools that are being used are becoming almost like the DNA of their engineering organization—everyone is starting to think a bit like a systems engineer, even in their normal job. So, by doing that, you’ve changed your whole organization. You don’t have to rely on a super specialized group of systems engineers to manage that process. Everybody is a stakeholder in that process. The tools and the ecosystem that you use to do systems engineering has a very large role in helping with that problem.
Laurel: Staying on that idea of simulation and artificial intelligence, that is certainly something that needs a lot of data, a lot of engineering to solve these really large problems. How many times do you go to the moon and back when you test out an autonomous vehicle? Hundreds, right? So, you need an enormous amount of data to be able to run the simulation or the models. Could you explain a bit more about how simulation—or even the concept of digital twin, which is creating a digital online environment to mimic what you would be actually building in the field—how does that fit into systems engineering?
Dale: It plays a very big part. It’s almost at the center of it. We often think about systems engineering in the context of requirements, system modeling, and then the verification processes to demonstrate that you’ve satisfied those requirements. That’s a classic closed-loop process of systems engineering, but simulation becomes a very critical tool towards being able to develop the architectures of your product and optimize those products. You can now look at thousands of options. You can run different tests. So, it plays a very big role in helping to define your product upfront.
Then, as you start your verification process, because you have the simulation tools to evaluate the performance of your product in many different configurations, you can identify design changes before you start building a product and before you start testing it. It plays a key role in the definition of the architecture, then the definition of the product, and then finally, the verification of the product. It helps optimize your processes that are being used to develop a new product.
Laurel: Nand, how does that help safety, when you can use simulation or digital twins, or just have more data to make these vehicles more safe?
Nand: Simulation forms the foundation of delivering a digital twin—or systems engineering, in my mind. So, with the simulation in the upfront phases, you can make the right architecture selections, and then move on into the detailed designs. It allows you to explore the space for optimization in delivering that solution. When you combine that with the physical representation of the same simulations and you bring these two things together, that’s how you increase the confidence in your simulation and the physical test results, which is called a CAE test correlation. That helps deliver the systems engineering. So, you could say simulation, digital twin, they go hand in hand in delivering or enabling systems engineering to go from end to end.
Laurel: So, Nand, how does systems engineering help scale product development and/or create this industrial efficiency? What is the return on investment?
Nand: That’s interesting. The industrial efficiency is one of the biggest end deliverables, how you monetize all these investments. I’ll use a couple of examples you asked about earlier. First, how do you deliver a safe vehicle? When you have a lot of simulations done, one of the goals is to reduce the number of physical prototypes built so that you can rely on that simulation. By definition, this is cheaper because you’re not consuming parts and machinery to build those prototypes, and that’s a big thing in the automotive industry. At the same time, you are doing a lot of innovation. There are some things which have not been done in a physical testing environment, so you have to go hand in hand and do some CAE correlation to build confidence. After that point, you’re generating another set of data through simulation. Now, in your next program or the next iteration of the design, you’re a lot more efficient.
Let me take that even further: how does artificial intelligence along with this massive simulation data fit in? There are a lot of cases where you take the simulation data, and through machine learning, you train the algorithms on the outcome of that particular simulation. So, if you’re doing an aerodynamic analysis and looking at a coefficient of drag, it’s intensive from a computational standpoint. Sometimes, those run up to five days to get results. If you’ve trained your algorithms through machine learning and artificial intelligence, you can keep building your database, for given test conditions, on what the results would be. At the end, when you have another new design scenario, you don’t have to do those five-day-long simulations. You put it through those algorithms, and that gives you the results within a matter of minutes. You can see a huge efficiency, both in terms of time it takes to do it and also the computing, which lowers the cost of all those things. That’s how you add on to the return on investments and expand your product development. You scale product development for multiple perspectives by doing more with less, with fewer people because with simulations and all these technologies combined, you can do the same amount of work. Or you could save with the same number of people by pushing through more products. In the automotive industry, you have simultaneously, sometimes, up to 20 programs running, and you can be more efficient.
Laurel: Dale, when we talk about return on investment and aircraft for aerospace defense, we’re talking about investing in a system and hardware that can last for years. An airplane is not replaced within a year. It needs to last a long time. How does ROI affect the way that people think about that with systems engineering?
Dale: That’s a great question. Some of the comments Nand made captured a lot of that very well. I usually think about this in two ways. One is that, when you think about a program, and in aerospace, it’s going through the development program, you’re working with large teams. You look at the budgets that are used for some of these programs, and they might spend 10 million, 20 million, maybe even $100 million a month. As part of that funding, they’re going through the certification process. If you can use simulation to avoid a month or two of delay, that’s a significant amount of money. A lot of times, if you only had a handful of simulation people working on this problem, the ROI can be 10, 20, 30, 40x. It’s a pretty amazing savings when you go through the process, or maybe it’s a pretty amazing cost avoidance.
The other piece of it, that you mentioned, is being able to support these programs over a 50, 60-year product development life cycle. Having the simulation place to be able to understand how the aircraft is performing once it’s out in the field, and by updating the digital twin, the simulation, you’re able to optimize maintenance cycles, which can be a huge cost savings for the operators. Again, the ROI may be in multiples of 10 or 20 on some of that. Sometimes, those costs are hidden, but it’s significant savings.
Then, when you want to upgrade or add new capabilities, because you have that digital twin and you have the simulation in place, you have already done the systems engineering work. It’s easier to integrate and bring new capabilities to the customer. You continue to add value throughout the entire product lifetime. So, the ROI is significant with a lot of these tools and goes beyond the first time you simulate and start designing the vehicle. It pays dividends throughout the entire product life cycle.
Laurel: So, Nand, earlier you mentioned smart cities and that systems engineering approaches could be extended to many different problem types in smart cities. How do you think systems engineering will help further invention and innovation?
Nand: In a smart city, your system has become the city and the vehicle in the city, as an example. Your definition of system has moved from a vehicle to the flow of traffic in the city, how the traffic lights operate in the city, and you can continue to extend that into other aspects of building management, as an example, into the smart city environment. In the autonomous vehicle case, the vehicles will be moving on their own without a driver, so that is part of the city. They have to work with the entire city infrastructure, the entire city traffic systems, the traffic controllers, and the autonomous vehicle. So, it becomes a totally different business case than what we have today. All these things are continuing to allow innovation, both at the technical level as well as at a business model level. As a result of autonomy and autonomous vehicle deployment, new business models are being formed. Whether it’s sharing the vehicle itself or delivering the goods or ride-sharing, that’s what I mean by continuing to innovate around what makes sense and how we can monetize and companies can be profitable.
In terms of the technical side of the business, connectivity is a big piece of it. As consumer trends like people watching Netflix on their phones at home, when they move into their car to go somewhere, they want continuity. They want to continue to watch in the car audio video system. Connectivity allows for more ideas around product development.
The big one in the auto industry is over-the-air updates. So, the whole paradigm shift from having to get a new model of your car every few years to most of the car features being updated through software, allows your hardware to stay the same. You can buy new features without going to a dealership because those features are distributed through software, and they can be delivered over-the-air while the vehicle is parked at your home, or wherever. Again, we’ve expanded the definition of system. The system has become the software being pushed from the author of that software to the end consumer for their products.
Laurel: Dale, how do you feel about innovation and invention with systems engineering?
Dale: Everything we’ve been talking about here today around connected cities and connected cars and connected airplanes and EVTOLs, or air taxis in general, is amazing when you think about the business models we haven’t thought about yet. Something we dream about, at least in aerospace, is like going to the moon—with a system of systems approach and the ability of all the new tools now to be able to look at more options, you might look at a completely different set of how to get to the moon and live on the moon. Instead of a rocket that’s launching, and then you transfer into a lunar lander, and you think about how the Apollo missions were set up, there was a lot of optimization that went into that, but now, you can look at it through the lens of completely different models.
As we start thinking about how we’re using energy around the world and how we’re working toward a more sustainable future, and as smart cities become more and more connected, how are you using energy effectively for your transportation? How are you using it more effectively with your power generation— when the sun gets at the hottest point of the day and you need to have air conditioning, how do you make buildings smarter so that, when there are fewer people in the building, the building can regulate the temperature to save electricity?
There are so many possibilities to think about how we’re using the resources we have and connecting people together better. There are going to be a lot of opportunities as people start to connect all of these devices together, to really become much more aware of our surroundings and how we’re interacting with cities and other people. I’m excited about it.
Laurel: Excellent. Nand and Dale, thank you so much for joining me today on the Business Lab.
Dale: It was great to be here, and I enjoyed the conversation today. Thank you.
Nand: Thank you, again. I enjoyed the conversation as well.
Laurel: That was Nand Kochhar, and Dale Tutt, from Siemens Software, whom I spoke with from Cambridge, Massachusetts, the home of MIT and MIT Technology Review, overlooking the Charles River. That’s it for this episode of Business Lab. I’m your host, Laurel Ruma. I’m the Director of Insights, the custom publishing division of MIT Technology Review. We were founded in 1899 at the Massachusetts Institute of Technology, and you could find us in prints on the web and at events each year around the world. For more information about us and the show, please check out our website at technologyreview.com. This show is available wherever you get your podcasts. If you enjoyed this episode, we hope you’ll take a moment to rate and review us. Business Lab is a production of MIT Technology Review. This episode was produced by Collective Next. Thanks for listening.
This podcast was produced by Insights, the custom content arm of MIT Technology Review. It was not written by MIT Technology Review’s editorial staff.
You may like
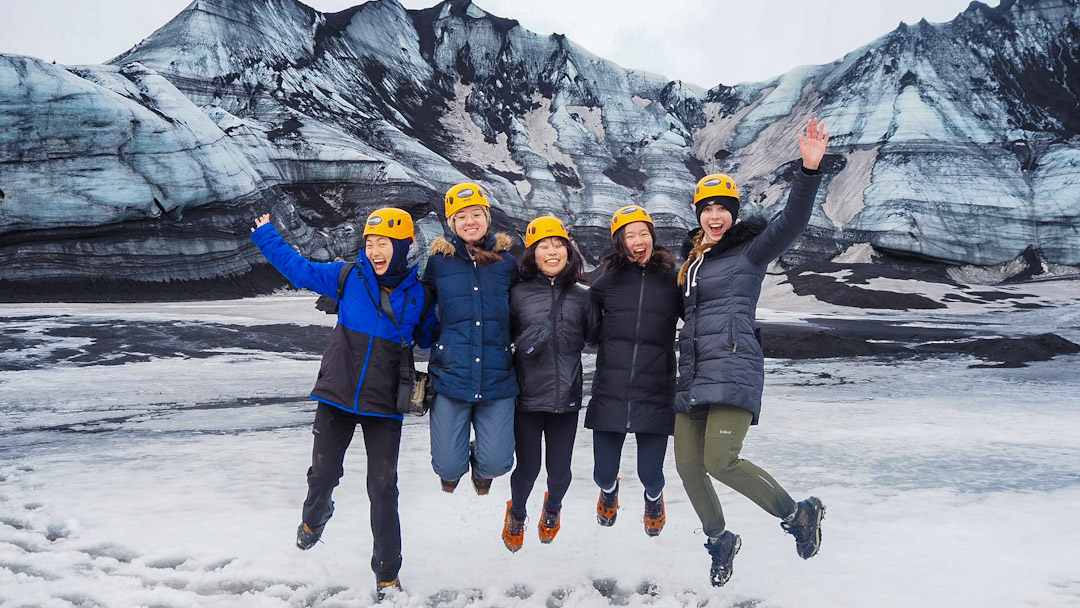
My senior spring in high school, I decided to defer my MIT enrollment by a year. I had always planned to take a gap year, but after receiving the silver tube in the mail and seeing all my college-bound friends plan out their classes and dorm decor, I got cold feet. Every time I mentioned my plans, I was met with questions like “But what about school?” and “MIT is cool with this?”
Yeah. MIT totally is. Postponing your MIT start date is as simple as clicking a checkbox.
COURTESY PHOTO
Now, having finished my first year of classes, I’m really grateful that I stuck with my decision to delay MIT, as I realized that having a full year of unstructured time is a gift. I could let my creative juices run. Pick up hobbies for fun. Do cool things like work at an AI startup and teach myself how to create latte art. My favorite part of the year, however, was backpacking across Europe. I traveled through Austria, Slovakia, Russia, Spain, France, the UK, Greece, Italy, Germany, Poland, Romania, and Hungary.
Moreover, despite my fear that I’d be losing a valuable year, traveling turned out to be the most productive thing I could have done with my time. I got to explore different cultures, meet new people from all over the world, and gain unique perspectives that I couldn’t have gotten otherwise. My travels throughout Europe allowed me to leave my comfort zone and expand my understanding of the greater human experience.
“In Iceland there’s less focus on hustle culture, and this relaxed approach to work-life balance ends up fostering creativity. This was a wild revelation to a bunch of MIT students.”
When I became a full-time student last fall, I realized that StartLabs, the premier undergraduate entrepreneurship club on campus, gives MIT undergrads a similar opportunity to expand their horizons and experience new things. I immediately signed up. At StartLabs, we host fireside chats and ideathons throughout the year. But our flagship event is our annual TechTrek over spring break. In previous years, StartLabs has gone on TechTrek trips to Germany, Switzerland, and Israel. On these fully funded trips, StartLabs members have visited and collaborated with industry leaders, incubators, startups, and academic institutions. They take these treks both to connect with the global startup sphere and to build closer relationships within the club itself.
Most important, however, the process of organizing the TechTrek is itself an expedited introduction to entrepreneurship. The trip is entirely planned by StartLabs members; we figure out travel logistics, find sponsors, and then discover ways to optimize our funding.
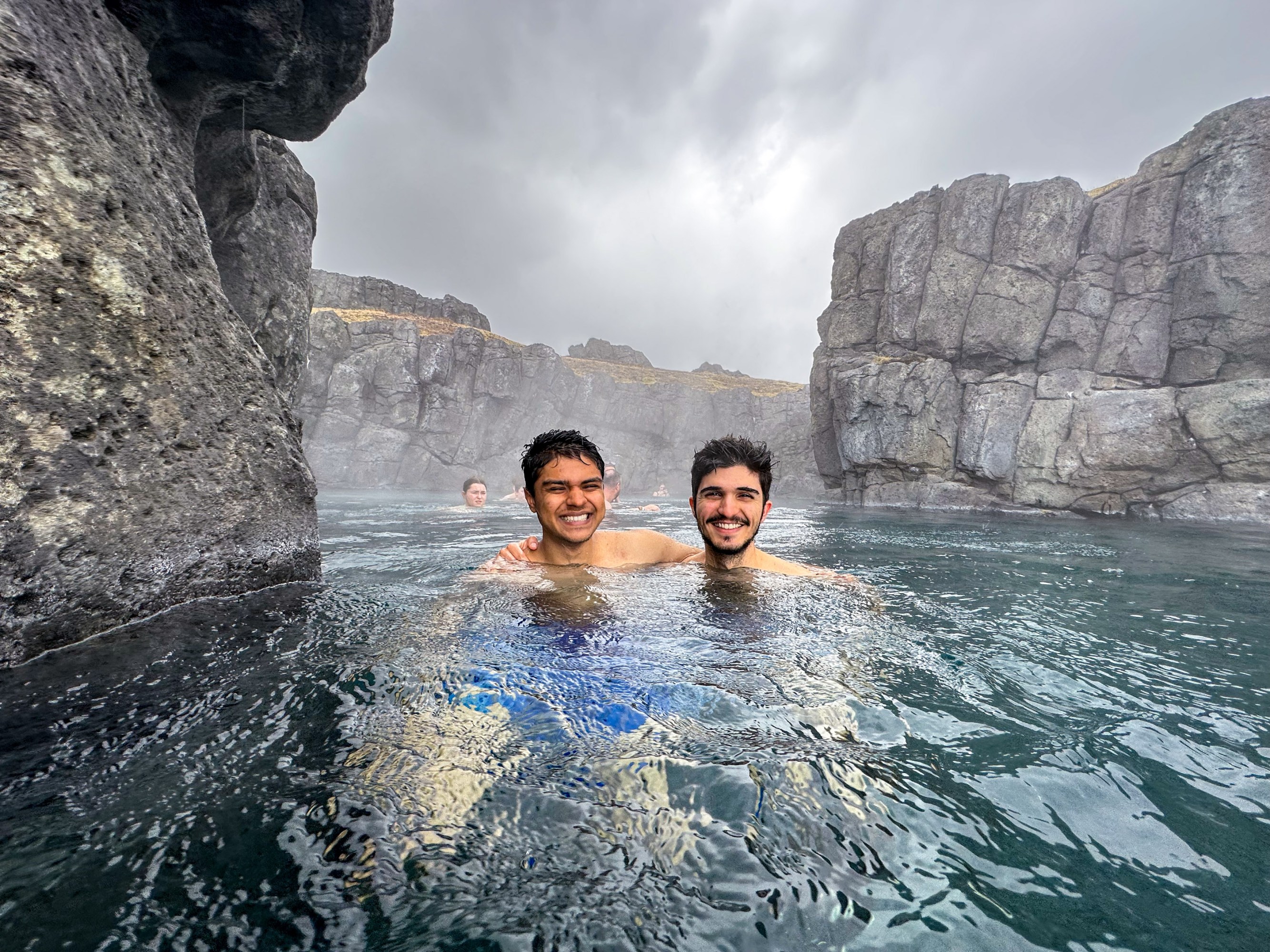
COURTESY PHOTO
In organizing this year’s trip to Iceland, we had to learn how to delegate roles to all the planners and how to maintain morale when making this trip a reality seemed to be an impossible task. We woke up extra early to take 6 a.m. calls with Icelandic founders and sponsors. We came up with options for different levels of sponsorship, used pattern recognition to deduce the email addresses of hundreds of potential contacts at organizations we wanted to visit, and all got scrappy with utilizing our LinkedIn connections.
And as any good entrepreneur must, we had to learn how to be lean and maximize our resources. To stretch our food budget, we planned all our incubator and company visits around lunchtime in hopes of getting fed, played human Tetris as we fit 16 people into a six-person Airbnb, and emailed grocery stores to get their nearly expired foods for a discount. We even made a deal with the local bus company to give us free tickets in exchange for a story post on our Instagram account.
Tech
The Download: spying keyboard software, and why boring AI is best
Published
9 months agoon
22 August 2023By
Terry Power
This is today’s edition of The Download, our weekday newsletter that provides a daily dose of what’s going on in the world of technology.
How ubiquitous keyboard software puts hundreds of millions of Chinese users at risk
For millions of Chinese people, the first software they download onto devices is always the same: a keyboard app. Yet few of them are aware that it may make everything they type vulnerable to spying eyes.
QWERTY keyboards are inefficient as many Chinese characters share the same latinized spelling. As a result, many switch to smart, localized keyboard apps to save time and frustration. Today, over 800 million Chinese people use third-party keyboard apps on their PCs, laptops, and mobile phones.
But a recent report by the Citizen Lab, a University of Toronto–affiliated research group, revealed that Sogou, one of the most popular Chinese keyboard apps, had a massive security loophole. Read the full story.
—Zeyi Yang
Why we should all be rooting for boring AI
Earlier this month, the US Department of Defense announced it is setting up a Generative AI Task Force, aimed at “analyzing and integrating” AI tools such as large language models across the department. It hopes they could improve intelligence and operational planning.
But those might not be the right use cases, writes our senior AI reporter Melissa Heikkila. Generative AI tools, such as language models, are glitchy and unpredictable, and they make things up. They also have massive security vulnerabilities, privacy problems, and deeply ingrained biases.
Applying these technologies in high-stakes settings could lead to deadly accidents where it’s unclear who or what should be held responsible, or even why the problem occurred. The DoD’s best bet is to apply generative AI to more mundane things like Excel, email, or word processing. Read the full story.
This story is from The Algorithm, Melissa’s weekly newsletter giving you the inside track on all things AI. Sign up to receive it in your inbox every Monday.
The ice cores that will let us look 1.5 million years into the past
To better understand the role atmospheric carbon dioxide plays in Earth’s climate cycles, scientists have long turned to ice cores drilled in Antarctica, where snow layers accumulate and compact over hundreds of thousands of years, trapping samples of ancient air in a lattice of bubbles that serve as tiny time capsules.
By analyzing those cores, scientists can connect greenhouse-gas concentrations with temperatures going back 800,000 years. Now, a new European-led initiative hopes to eventually retrieve the oldest core yet, dating back 1.5 million years. But that impressive feat is still only the first step. Once they’ve done that, they’ll have to figure out how they’re going to extract the air from the ice. Read the full story.
—Christian Elliott
This story is from the latest edition of our print magazine, set to go live tomorrow. Subscribe today for as low as $8/month to ensure you receive full access to the new Ethics issue and in-depth stories on experimental drugs, AI assisted warfare, microfinance, and more.
The must-reads
I’ve combed the internet to find you today’s most fun/important/scary/fascinating stories about technology.
1 How AI got dragged into the culture wars
Fears about ‘woke’ AI fundamentally misunderstand how it works. Yet they’re gaining traction. (The Guardian)
+ Why it’s impossible to build an unbiased AI language model. (MIT Technology Review)
2 Researchers are racing to understand a new coronavirus variant
It’s unlikely to be cause for concern, but it shows this virus still has plenty of tricks up its sleeve. (Nature)
+ Covid hasn’t entirely gone away—here’s where we stand. (MIT Technology Review)
+ Why we can’t afford to stop monitoring it. (Ars Technica)
3 How Hilary became such a monster storm
Much of it is down to unusually hot sea surface temperatures. (Wired $)
+ The era of simultaneous climate disasters is here to stay. (Axios)
+ People are donning cooling vests so they can work through the heat. (Wired $)
4 Brain privacy is set to become important
Scientists are getting better at decoding our brain data. It’s surely only a matter of time before others want a peek. (The Atlantic $)
+ How your brain data could be used against you. (MIT Technology Review)
5 How Nvidia built such a big competitive advantage in AI chips
Today it accounts for 70% of all AI chip sales—and an even greater share for training generative models. (NYT $)
+ The chips it’s selling to China are less effective due to US export controls. (Ars Technica)
+ These simple design rules could turn the chip industry on its head. (MIT Technology Review)
6 Inside the complex world of dissociative identity disorder on TikTok
Reducing stigma is great, but doctors fear people are self-diagnosing or even imitating the disorder. (The Verge)
7 What TikTok might have to give up to keep operating in the US
This shows just how hollow the authorities’ purported data-collection concerns really are. (Forbes)
8 Soldiers in Ukraine are playing World of Tanks on their phones
It’s eerily similar to the war they are themselves fighting, but they say it helps them to dissociate from the horror. (NYT $)
9 Conspiracy theorists are sharing mad ideas on what causes wildfires
But it’s all just a convoluted way to try to avoid having to tackle climate change. (Slate $)
10 Christie’s accidentally leaked the location of tons of valuable art
Seemingly thanks to the metadata that often automatically attaches to smartphone photos. (WP $)
Quote of the day
“Is it going to take people dying for something to move forward?”
—An anonymous air traffic controller warns that staffing shortages in their industry, plus other factors, are starting to threaten passenger safety, the New York Times reports.
The big story
Inside effective altruism, where the far future counts a lot more than the present

October 2022
Since its birth in the late 2000s, effective altruism has aimed to answer the question “How can those with means have the most impact on the world in a quantifiable way?”—and supplied methods for calculating the answer.
It’s no surprise that effective altruisms’ ideas have long faced criticism for reflecting white Western saviorism, alongside an avoidance of structural problems in favor of abstract math. And as believers pour even greater amounts of money into the movement’s increasingly sci-fi ideals, such charges are only intensifying. Read the full story.
—Rebecca Ackermann
We can still have nice things
A place for comfort, fun and distraction in these weird times. (Got any ideas? Drop me a line or tweet ’em at me.)
+ Watch Andrew Scott’s electrifying reading of the 1965 commencement address ‘Choose One of Five’ by Edith Sampson.
+ Here’s how Metallica makes sure its live performances ROCK. ($)
+ Cannot deal with this utterly ludicrous wooden vehicle.
+ Learn about a weird and wonderful new instrument called a harpejji.
Tech
Why we should all be rooting for boring AI
Published
9 months agoon
22 August 2023By
Terry Power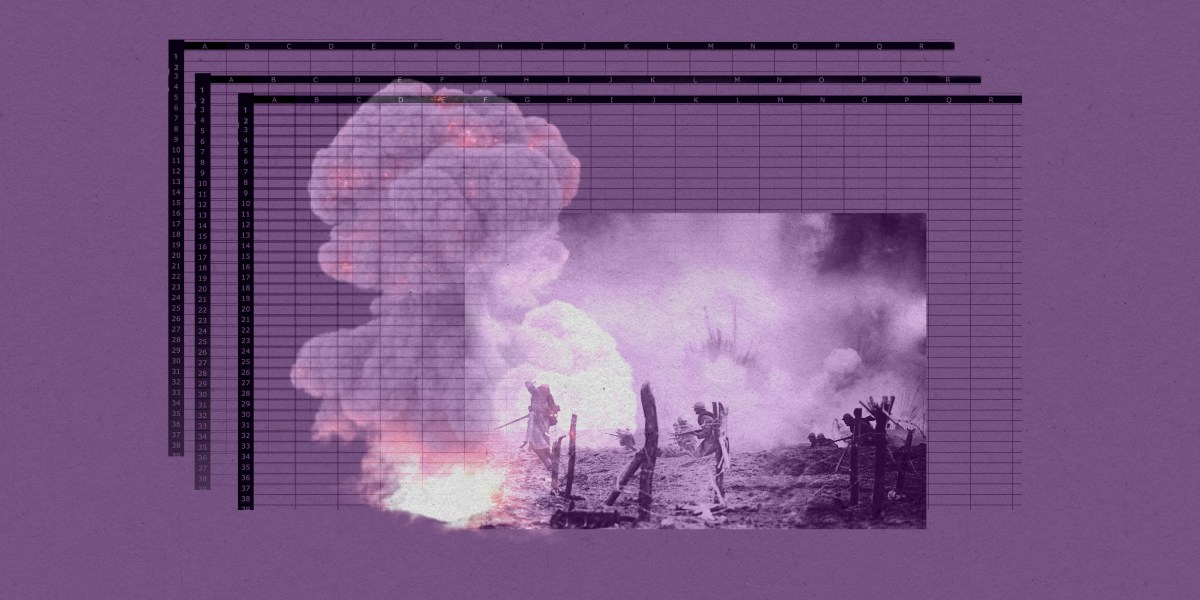
This story originally appeared in The Algorithm, our weekly newsletter on AI. To get stories like this in your inbox first, sign up here.
I’m back from a wholesome week off picking blueberries in a forest. So this story we published last week about the messy ethics of AI in warfare is just the antidote, bringing my blood pressure right back up again.
Arthur Holland Michel does a great job looking at the complicated and nuanced ethical questions around warfare and the military’s increasing use of artificial-intelligence tools. There are myriad ways AI could fail catastrophically or be abused in conflict situations, and there don’t seem to be any real rules constraining it yet. Holland Michel’s story illustrates how little there is to hold people accountable when things go wrong.
Last year I wrote about how the war in Ukraine kick-started a new boom in business for defense AI startups. The latest hype cycle has only added to that, as companies—and now the military too—race to embed generative AI in products and services.
Earlier this month, the US Department of Defense announced it is setting up a Generative AI Task Force, aimed at “analyzing and integrating” AI tools such as large language models across the department.
The department sees tons of potential to “improve intelligence, operational planning, and administrative and business processes.”
But Holland Michel’s story highlights why the first two use cases might be a bad idea. Generative AI tools, such as language models, are glitchy and unpredictable, and they make things up. They also have massive security vulnerabilities, privacy problems, and deeply ingrained biases.
Applying these technologies in high-stakes settings could lead to deadly accidents where it’s unclear who or what should be held responsible, or even why the problem occurred. Everyone agrees that humans should make the final call, but that is made harder by technology that acts unpredictably, especially in fast-moving conflict situations.
Some worry that the people lowest on the hierarchy will pay the highest price when things go wrong: “In the event of an accident—regardless of whether the human was wrong, the computer was wrong, or they were wrong together—the person who made the ‘decision’ will absorb the blame and protect everyone else along the chain of command from the full impact of accountability,” Holland Michel writes.
The only ones who seem likely to face no consequences when AI fails in war are the companies supplying the technology.
It helps companies when the rules the US has set to govern AI in warfare are mere recommendations, not laws. That makes it really hard to hold anyone accountable. Even the AI Act, the EU’s sweeping upcoming regulation for high-risk AI systems, exempts military uses, which arguably are the highest-risk applications of them all.
While everyone is looking for exciting new uses for generative AI, I personally can’t wait for it to become boring.
Amid early signs that people are starting to lose interest in the technology, companies might find that these sorts of tools are better suited for mundane, low-risk applications than solving humanity’s biggest problems.
Applying AI in, for example, productivity software such as Excel, email, or word processing might not be the sexiest idea, but compared to warfare it’s a relatively low-stakes application, and simple enough to have the potential to actually work as advertised. It could help us do the tedious bits of our jobs faster and better.
Boring AI is unlikely to break as easily and, most important, won’t kill anyone. Hopefully, soon we’ll forget we’re interacting with AI at all. (It wasn’t that long ago when machine translation was an exciting new thing in AI. Now most people don’t even think about its role in powering Google Translate.)
That’s why I’m more confident that organizations like the DoD will find success applying generative AI in administrative and business processes.
Boring AI is not morally complex. It’s not magic. But it works.
Deeper Learning
AI isn’t great at decoding human emotions. So why are regulators targeting the tech?
Amid all the chatter about ChatGPT, artificial general intelligence, and the prospect of robots taking people’s jobs, regulators in the EU and the US have been ramping up warnings against AI and emotion recognition. Emotion recognition is the attempt to identify a person’s feelings or state of mind using AI analysis of video, facial images, or audio recordings.
But why is this a top concern? Western regulators are particularly concerned about China’s use of the technology, and its potential to enable social control. And there’s also evidence that it simply does not work properly. Tate Ryan-Mosley dissected the thorny questions around the technology in last week’s edition of The Technocrat, our weekly newsletter on tech policy.
Bits and Bytes
Meta is preparing to launch free code-generating software
A version of its new LLaMA 2 language model that is able to generate programming code will pose a stiff challenge to similar proprietary code-generating programs from rivals such as OpenAI, Microsoft, and Google. The open-source program is called Code Llama, and its launch is imminent, according to The Information. (The Information)
OpenAI is testing GPT-4 for content moderation
Using the language model to moderate online content could really help alleviate the mental toll content moderation takes on humans. OpenAI says it’s seen some promising first results, although the tech does not outperform highly trained humans. A lot of big, open questions remain, such as whether the tool can be attuned to different cultures and pick up context and nuance. (OpenAI)
Google is working on an AI assistant that offers life advice
The generative AI tools could function as a life coach, offering up ideas, planning instructions, and tutoring tips. (The New York Times)
Two tech luminaries have quit their jobs to build AI systems inspired by bees
Sakana, a new AI research lab, draws inspiration from the animal kingdom. Founded by two prominent industry researchers and former Googlers, the company plans to make multiple smaller AI models that work together, the idea being that a “swarm” of programs could be as powerful as a single large AI model. (Bloomberg)