Tech
Getting value from your data shouldn’t be this hard
Published
3 years agoon
By
Terry Power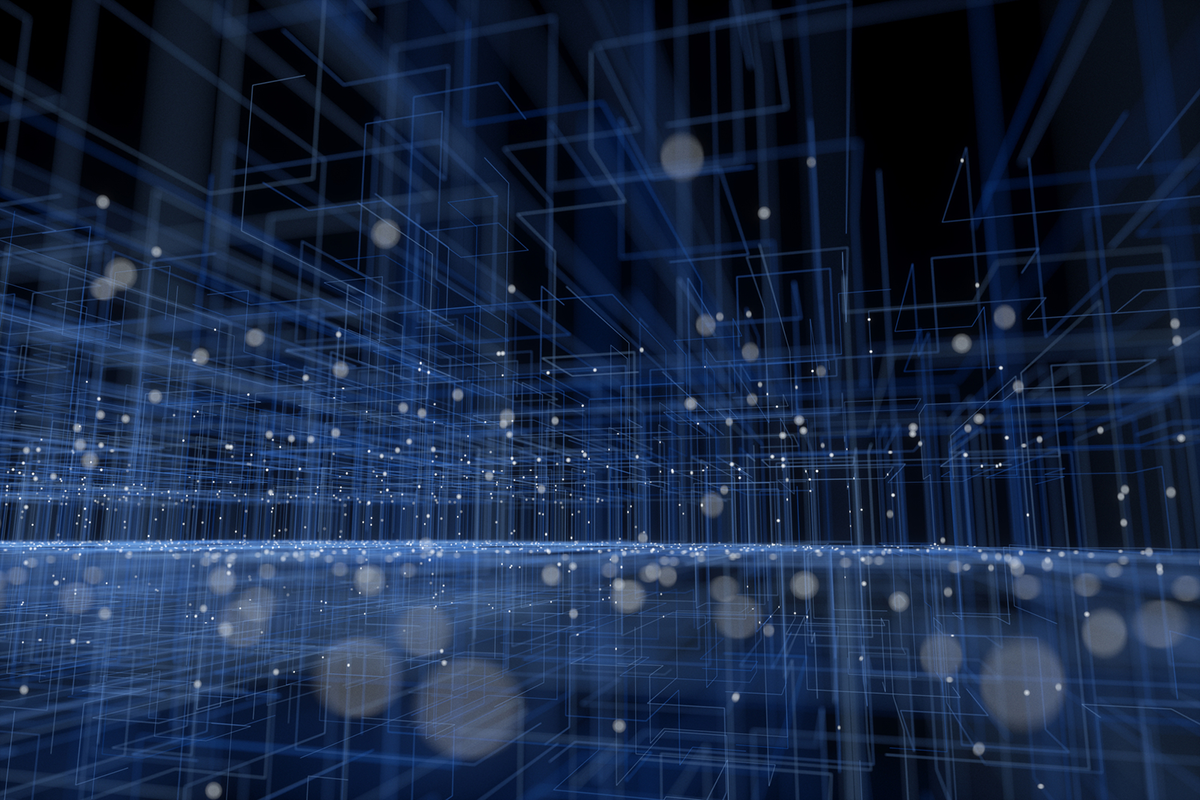
The potential impact of the ongoing worldwide data explosion continues to excite the imagination. A 2018 report estimated that every second of every day, every person produces 1.7 MB of data on average—and annual data creation has more than doubled since then and is projected to more than double again by 2025. A report from McKinsey Global Institute estimates that skillful uses of big data could generate an additional $3 trillion in economic activity, enabling applications as diverse as self-driving cars, personalized health care, and traceable food supply chains.
But adding all this data to the system is also creating confusion about how to find it, use it, manage it, and legally, securely, and efficiently share it. Where did a certain dataset come from? Who owns what? Who’s allowed to see certain things? Where does it reside? Can it be shared? Can it be sold? Can people see how it was used?
As data’s applications grow and become more ubiquitous, producers, consumers, and owners and stewards of data are finding that they don’t have a playbook to follow. Consumers want to connect to data they trust so they can make the best possible decisions. Producers need tools to share their data safely with those who need it. But technology platforms fall short, and there are no real common sources of truth to connect both sides.
How do we find data? When should we move it?
In a perfect world, data would flow freely like a utility accessible to all. It could be packaged up and sold like raw materials. It could be viewed easily, without complications, by anyone authorized to see it. Its origins and movements could be tracked, removing any concerns about nefarious uses somewhere along the line.
Today’s world, of course, does not operate this way. The massive data explosion has created a long list of issues and opportunities that make it tricky to share chunks of information.
With data being created nearly everywhere within and outside of an organization, the first challenge is identifying what is being gathered and how to organize it so it can be found.
A lack of transparency and sovereignty over stored and processed data and infrastructure opens up trust issues. Today, moving data to centralized locations from multiple technology stacks is expensive and inefficient. The absence of open metadata standards and widely accessible application programming interfaces can make it hard to access and consume data. The presence of sector-specific data ontologies can make it hard for people outside the sector to benefit from new sources of data. Multiple stakeholders and difficulty accessing existing data services can make it hard to share without a governance model.
Europe is taking the lead
Despite the issues, data-sharing projects are being undertaken on a grand scale. One that’s backed by the European Union and a nonprofit group is creating an interoperable data exchange called Gaia-X, where businesses can share data under the protection of strict European data privacy laws. The exchange is envisioned as a vessel to share data across industries and a repository for information about data services around artificial intelligence (AI), analytics, and the internet of things.
Hewlett Packard Enterprise recently announced a solution framework to support companies, service providers, and public organizations’ participation in Gaia-X. The dataspaces platform, which is currently in development and based on open standards and cloud native, democratizes access to data, data analytics, and AI by making them more accessible to domain experts and common users. It provides a place where experts from domain areas can more easily identify trustworthy datasets and securely perform analytics on operational data—without always requiring the costly movement of data to centralized locations.
By using this framework to integrate complex data sources across IT landscapes, enterprises will be able to provide data transparency at scale, so everyone—whether a data scientist or not—knows what data they have, how to access it, and how to use it in real time.
Data-sharing initiatives are also on the top of enterprises’ agendas. One important priority enterprises face is the vetting of data that’s being used to train internal AI and machine learning models. AI and machine learning are already being used widely in enterprises and industry to drive ongoing improvements in everything from product development to recruiting to manufacturing. And we’re just getting started. IDC projects the global AI market will grow from $328 billion in 2021 to $554 billion in 2025.
To unlock AI’s true potential, governments and enterprises need to better understand the collective legacy of all the data that is driving these models. How do AI models make their decisions? Do they have bias? Are they trustworthy? Have untrustworthy individuals been able to access or change the data that an enterprise has trained its model against? Connecting data producers to data consumers more transparently and with greater efficiency can help answer some of these questions.
Building data maturity
Enterprises aren’t going to solve how to unlock all of their data overnight. But they can prepare themselves to take advantage of technologies and management concepts that help to create a data-sharing mentality. They can ensure that they’re developing the maturity to consume or share data strategically and effectively rather than doing it on an ad hoc basis.
Data producers can prepare for wider distribution of data by taking a series of steps. They need to understand where their data is and understand how they’re collecting it. Then, they need to make sure the people who consume the data have the ability to access the right sets of data at the right times. That’s the starting point.
Then comes the harder part. If a data producer has consumers—which can be inside or outside the organization—they have to connect to the data. That’s both an organizational and a technology challenge. Many organizations want governance over data sharing with other organizations. The democratization of data—at least being able to find it across organizations—is an organizational maturity issue. How do they handle that?
Companies that contribute to the auto industry actively share data with vendors, partners, and subcontractors. It takes a lot of parts—and a lot of coordination—to assemble a car. Partners readily share information on everything from engines to tires to web-enabled repair channels. Automotive dataspaces can serve upwards of 10,000 vendors. But in other industries, it might be more insular. Some large companies might not want to share sensitive information even within their own network of business units.
Creating a data mentality
Companies on either side of the consumer-producer continuum can advance their data-sharing mentality by asking themselves these strategic questions:
- If enterprises are building AI and machine learning solutions, where are the teams getting their data? How are they connecting to that data? And how do they track that history to ensure trustworthiness and provenance of data?
- If data has value to others, what is the monetization path the team is taking today to expand on that value, and how will it be governed?
- If a company is already exchanging or monetizing data, can it authorize a broader set of services on multiple platforms—on premises and in the cloud?
- For organizations that need to share data with vendors, how is the coordination of those vendors to the same datasets and updates getting done today?
- Do producers want to replicate their data or force people to bring models to them? Datasets might be so large that they can’t be replicated. Should a company host software developers on its platform where its data is and move the models in and out?
- How can workers in a department that consumes data influence the practices of the upstream data producers within their organization?
Taking action
The data revolution is creating business opportunities—along with plenty of confusion about how to search for, collect, manage, and gain insights from that data in a strategic way. Data producers and data consumers are becoming more disconnected with each other. HPE is building a platform supporting both on-premises and public cloud, using open source as the foundation and solutions like HPE Ezmeral Software Platform to provide the common ground both sides need to make the data revolution work for them.
Read the original article on Enterprise.nxt.
This content was produced by Hewlett Packard Enterprise. It was not written by MIT Technology Review’s editorial staff.
You may like
-
Why is it so hard to create new types of pain relievers?
-
Decoding the data of the Chinese mpox outbreak
-
How tech companies got access to our tax data
-
Universal Data Intelligence: The Backbone for AI
-
Making data matter in real time
-
China’s Omission Of Cremation Data Raises Questions About COVID-19 Impact
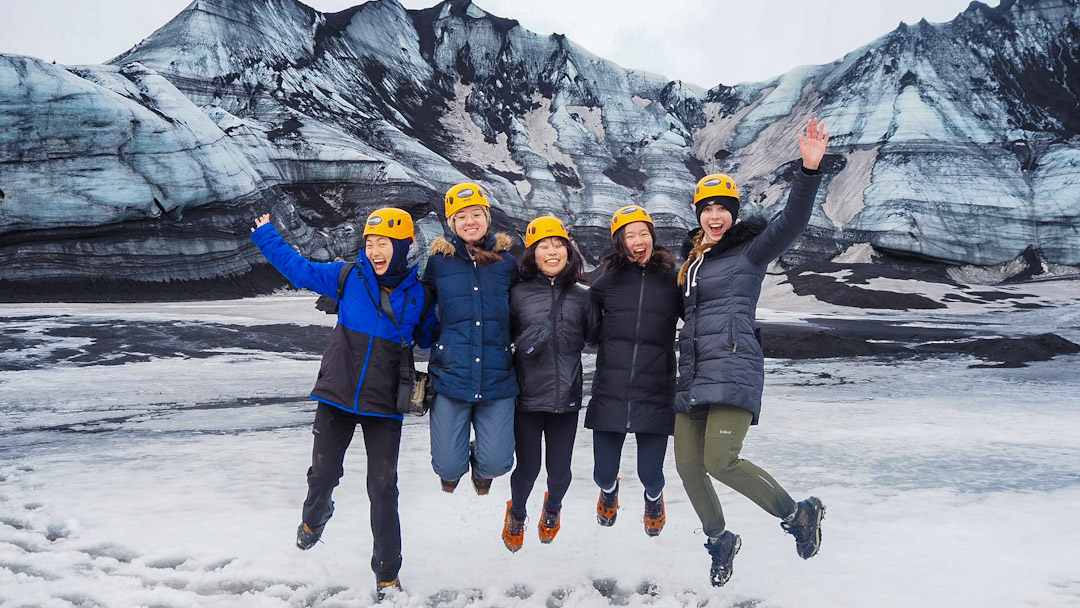
My senior spring in high school, I decided to defer my MIT enrollment by a year. I had always planned to take a gap year, but after receiving the silver tube in the mail and seeing all my college-bound friends plan out their classes and dorm decor, I got cold feet. Every time I mentioned my plans, I was met with questions like “But what about school?” and “MIT is cool with this?”
Yeah. MIT totally is. Postponing your MIT start date is as simple as clicking a checkbox.
COURTESY PHOTO
Now, having finished my first year of classes, I’m really grateful that I stuck with my decision to delay MIT, as I realized that having a full year of unstructured time is a gift. I could let my creative juices run. Pick up hobbies for fun. Do cool things like work at an AI startup and teach myself how to create latte art. My favorite part of the year, however, was backpacking across Europe. I traveled through Austria, Slovakia, Russia, Spain, France, the UK, Greece, Italy, Germany, Poland, Romania, and Hungary.
Moreover, despite my fear that I’d be losing a valuable year, traveling turned out to be the most productive thing I could have done with my time. I got to explore different cultures, meet new people from all over the world, and gain unique perspectives that I couldn’t have gotten otherwise. My travels throughout Europe allowed me to leave my comfort zone and expand my understanding of the greater human experience.
“In Iceland there’s less focus on hustle culture, and this relaxed approach to work-life balance ends up fostering creativity. This was a wild revelation to a bunch of MIT students.”
When I became a full-time student last fall, I realized that StartLabs, the premier undergraduate entrepreneurship club on campus, gives MIT undergrads a similar opportunity to expand their horizons and experience new things. I immediately signed up. At StartLabs, we host fireside chats and ideathons throughout the year. But our flagship event is our annual TechTrek over spring break. In previous years, StartLabs has gone on TechTrek trips to Germany, Switzerland, and Israel. On these fully funded trips, StartLabs members have visited and collaborated with industry leaders, incubators, startups, and academic institutions. They take these treks both to connect with the global startup sphere and to build closer relationships within the club itself.
Most important, however, the process of organizing the TechTrek is itself an expedited introduction to entrepreneurship. The trip is entirely planned by StartLabs members; we figure out travel logistics, find sponsors, and then discover ways to optimize our funding.
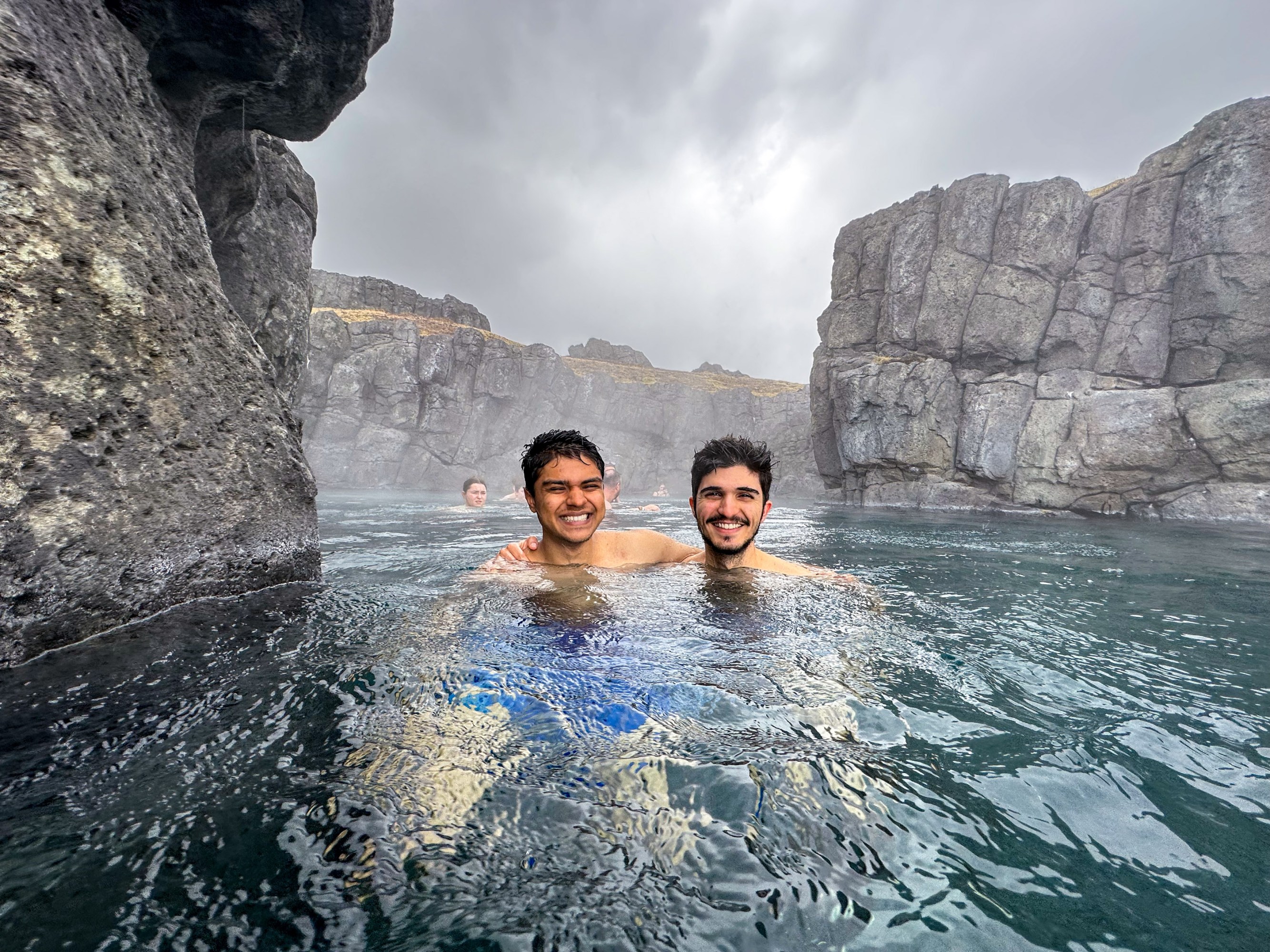
COURTESY PHOTO
In organizing this year’s trip to Iceland, we had to learn how to delegate roles to all the planners and how to maintain morale when making this trip a reality seemed to be an impossible task. We woke up extra early to take 6 a.m. calls with Icelandic founders and sponsors. We came up with options for different levels of sponsorship, used pattern recognition to deduce the email addresses of hundreds of potential contacts at organizations we wanted to visit, and all got scrappy with utilizing our LinkedIn connections.
And as any good entrepreneur must, we had to learn how to be lean and maximize our resources. To stretch our food budget, we planned all our incubator and company visits around lunchtime in hopes of getting fed, played human Tetris as we fit 16 people into a six-person Airbnb, and emailed grocery stores to get their nearly expired foods for a discount. We even made a deal with the local bus company to give us free tickets in exchange for a story post on our Instagram account.
Tech
The Download: spying keyboard software, and why boring AI is best
Published
1 year agoon
22 August 2023By
Terry Power
This is today’s edition of The Download, our weekday newsletter that provides a daily dose of what’s going on in the world of technology.
How ubiquitous keyboard software puts hundreds of millions of Chinese users at risk
For millions of Chinese people, the first software they download onto devices is always the same: a keyboard app. Yet few of them are aware that it may make everything they type vulnerable to spying eyes.
QWERTY keyboards are inefficient as many Chinese characters share the same latinized spelling. As a result, many switch to smart, localized keyboard apps to save time and frustration. Today, over 800 million Chinese people use third-party keyboard apps on their PCs, laptops, and mobile phones.
But a recent report by the Citizen Lab, a University of Toronto–affiliated research group, revealed that Sogou, one of the most popular Chinese keyboard apps, had a massive security loophole. Read the full story.
—Zeyi Yang
Why we should all be rooting for boring AI
Earlier this month, the US Department of Defense announced it is setting up a Generative AI Task Force, aimed at “analyzing and integrating” AI tools such as large language models across the department. It hopes they could improve intelligence and operational planning.
But those might not be the right use cases, writes our senior AI reporter Melissa Heikkila. Generative AI tools, such as language models, are glitchy and unpredictable, and they make things up. They also have massive security vulnerabilities, privacy problems, and deeply ingrained biases.
Applying these technologies in high-stakes settings could lead to deadly accidents where it’s unclear who or what should be held responsible, or even why the problem occurred. The DoD’s best bet is to apply generative AI to more mundane things like Excel, email, or word processing. Read the full story.
This story is from The Algorithm, Melissa’s weekly newsletter giving you the inside track on all things AI. Sign up to receive it in your inbox every Monday.
The ice cores that will let us look 1.5 million years into the past
To better understand the role atmospheric carbon dioxide plays in Earth’s climate cycles, scientists have long turned to ice cores drilled in Antarctica, where snow layers accumulate and compact over hundreds of thousands of years, trapping samples of ancient air in a lattice of bubbles that serve as tiny time capsules.
By analyzing those cores, scientists can connect greenhouse-gas concentrations with temperatures going back 800,000 years. Now, a new European-led initiative hopes to eventually retrieve the oldest core yet, dating back 1.5 million years. But that impressive feat is still only the first step. Once they’ve done that, they’ll have to figure out how they’re going to extract the air from the ice. Read the full story.
—Christian Elliott
This story is from the latest edition of our print magazine, set to go live tomorrow. Subscribe today for as low as $8/month to ensure you receive full access to the new Ethics issue and in-depth stories on experimental drugs, AI assisted warfare, microfinance, and more.
The must-reads
I’ve combed the internet to find you today’s most fun/important/scary/fascinating stories about technology.
1 How AI got dragged into the culture wars
Fears about ‘woke’ AI fundamentally misunderstand how it works. Yet they’re gaining traction. (The Guardian)
+ Why it’s impossible to build an unbiased AI language model. (MIT Technology Review)
2 Researchers are racing to understand a new coronavirus variant
It’s unlikely to be cause for concern, but it shows this virus still has plenty of tricks up its sleeve. (Nature)
+ Covid hasn’t entirely gone away—here’s where we stand. (MIT Technology Review)
+ Why we can’t afford to stop monitoring it. (Ars Technica)
3 How Hilary became such a monster storm
Much of it is down to unusually hot sea surface temperatures. (Wired $)
+ The era of simultaneous climate disasters is here to stay. (Axios)
+ People are donning cooling vests so they can work through the heat. (Wired $)
4 Brain privacy is set to become important
Scientists are getting better at decoding our brain data. It’s surely only a matter of time before others want a peek. (The Atlantic $)
+ How your brain data could be used against you. (MIT Technology Review)
5 How Nvidia built such a big competitive advantage in AI chips
Today it accounts for 70% of all AI chip sales—and an even greater share for training generative models. (NYT $)
+ The chips it’s selling to China are less effective due to US export controls. (Ars Technica)
+ These simple design rules could turn the chip industry on its head. (MIT Technology Review)
6 Inside the complex world of dissociative identity disorder on TikTok
Reducing stigma is great, but doctors fear people are self-diagnosing or even imitating the disorder. (The Verge)
7 What TikTok might have to give up to keep operating in the US
This shows just how hollow the authorities’ purported data-collection concerns really are. (Forbes)
8 Soldiers in Ukraine are playing World of Tanks on their phones
It’s eerily similar to the war they are themselves fighting, but they say it helps them to dissociate from the horror. (NYT $)
9 Conspiracy theorists are sharing mad ideas on what causes wildfires
But it’s all just a convoluted way to try to avoid having to tackle climate change. (Slate $)
10 Christie’s accidentally leaked the location of tons of valuable art
Seemingly thanks to the metadata that often automatically attaches to smartphone photos. (WP $)
Quote of the day
“Is it going to take people dying for something to move forward?”
—An anonymous air traffic controller warns that staffing shortages in their industry, plus other factors, are starting to threaten passenger safety, the New York Times reports.
The big story
Inside effective altruism, where the far future counts a lot more than the present

October 2022
Since its birth in the late 2000s, effective altruism has aimed to answer the question “How can those with means have the most impact on the world in a quantifiable way?”—and supplied methods for calculating the answer.
It’s no surprise that effective altruisms’ ideas have long faced criticism for reflecting white Western saviorism, alongside an avoidance of structural problems in favor of abstract math. And as believers pour even greater amounts of money into the movement’s increasingly sci-fi ideals, such charges are only intensifying. Read the full story.
—Rebecca Ackermann
We can still have nice things
A place for comfort, fun and distraction in these weird times. (Got any ideas? Drop me a line or tweet ’em at me.)
+ Watch Andrew Scott’s electrifying reading of the 1965 commencement address ‘Choose One of Five’ by Edith Sampson.
+ Here’s how Metallica makes sure its live performances ROCK. ($)
+ Cannot deal with this utterly ludicrous wooden vehicle.
+ Learn about a weird and wonderful new instrument called a harpejji.
Tech
Why we should all be rooting for boring AI
Published
1 year agoon
22 August 2023By
Terry Power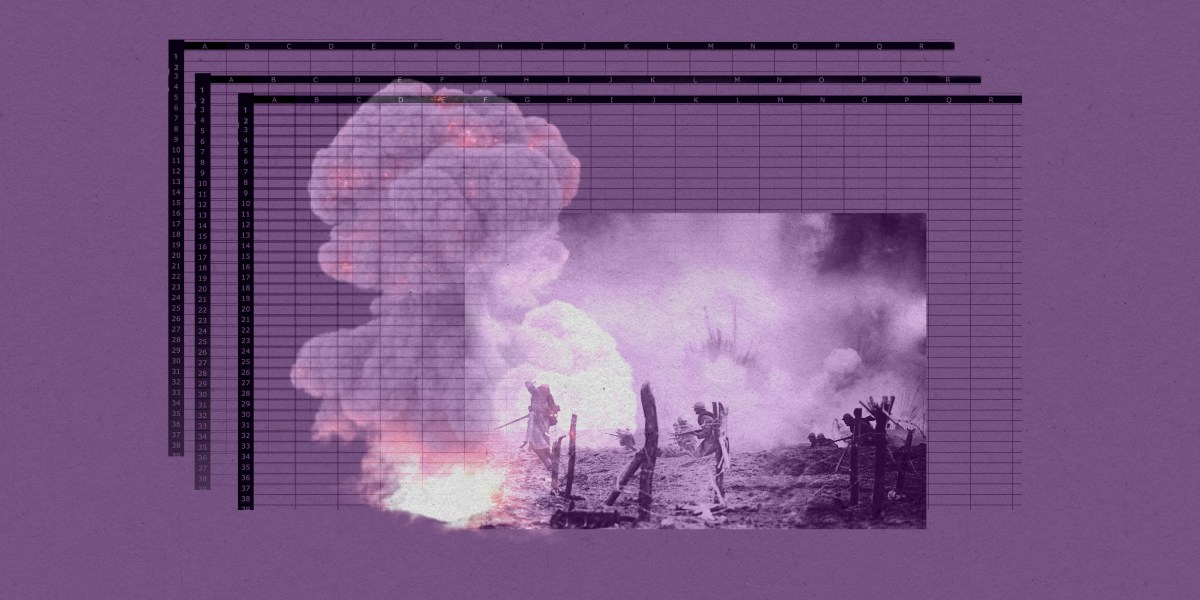
This story originally appeared in The Algorithm, our weekly newsletter on AI. To get stories like this in your inbox first, sign up here.
I’m back from a wholesome week off picking blueberries in a forest. So this story we published last week about the messy ethics of AI in warfare is just the antidote, bringing my blood pressure right back up again.
Arthur Holland Michel does a great job looking at the complicated and nuanced ethical questions around warfare and the military’s increasing use of artificial-intelligence tools. There are myriad ways AI could fail catastrophically or be abused in conflict situations, and there don’t seem to be any real rules constraining it yet. Holland Michel’s story illustrates how little there is to hold people accountable when things go wrong.
Last year I wrote about how the war in Ukraine kick-started a new boom in business for defense AI startups. The latest hype cycle has only added to that, as companies—and now the military too—race to embed generative AI in products and services.
Earlier this month, the US Department of Defense announced it is setting up a Generative AI Task Force, aimed at “analyzing and integrating” AI tools such as large language models across the department.
The department sees tons of potential to “improve intelligence, operational planning, and administrative and business processes.”
But Holland Michel’s story highlights why the first two use cases might be a bad idea. Generative AI tools, such as language models, are glitchy and unpredictable, and they make things up. They also have massive security vulnerabilities, privacy problems, and deeply ingrained biases.
Applying these technologies in high-stakes settings could lead to deadly accidents where it’s unclear who or what should be held responsible, or even why the problem occurred. Everyone agrees that humans should make the final call, but that is made harder by technology that acts unpredictably, especially in fast-moving conflict situations.
Some worry that the people lowest on the hierarchy will pay the highest price when things go wrong: “In the event of an accident—regardless of whether the human was wrong, the computer was wrong, or they were wrong together—the person who made the ‘decision’ will absorb the blame and protect everyone else along the chain of command from the full impact of accountability,” Holland Michel writes.
The only ones who seem likely to face no consequences when AI fails in war are the companies supplying the technology.
It helps companies when the rules the US has set to govern AI in warfare are mere recommendations, not laws. That makes it really hard to hold anyone accountable. Even the AI Act, the EU’s sweeping upcoming regulation for high-risk AI systems, exempts military uses, which arguably are the highest-risk applications of them all.
While everyone is looking for exciting new uses for generative AI, I personally can’t wait for it to become boring.
Amid early signs that people are starting to lose interest in the technology, companies might find that these sorts of tools are better suited for mundane, low-risk applications than solving humanity’s biggest problems.
Applying AI in, for example, productivity software such as Excel, email, or word processing might not be the sexiest idea, but compared to warfare it’s a relatively low-stakes application, and simple enough to have the potential to actually work as advertised. It could help us do the tedious bits of our jobs faster and better.
Boring AI is unlikely to break as easily and, most important, won’t kill anyone. Hopefully, soon we’ll forget we’re interacting with AI at all. (It wasn’t that long ago when machine translation was an exciting new thing in AI. Now most people don’t even think about its role in powering Google Translate.)
That’s why I’m more confident that organizations like the DoD will find success applying generative AI in administrative and business processes.
Boring AI is not morally complex. It’s not magic. But it works.
Deeper Learning
AI isn’t great at decoding human emotions. So why are regulators targeting the tech?
Amid all the chatter about ChatGPT, artificial general intelligence, and the prospect of robots taking people’s jobs, regulators in the EU and the US have been ramping up warnings against AI and emotion recognition. Emotion recognition is the attempt to identify a person’s feelings or state of mind using AI analysis of video, facial images, or audio recordings.
But why is this a top concern? Western regulators are particularly concerned about China’s use of the technology, and its potential to enable social control. And there’s also evidence that it simply does not work properly. Tate Ryan-Mosley dissected the thorny questions around the technology in last week’s edition of The Technocrat, our weekly newsletter on tech policy.
Bits and Bytes
Meta is preparing to launch free code-generating software
A version of its new LLaMA 2 language model that is able to generate programming code will pose a stiff challenge to similar proprietary code-generating programs from rivals such as OpenAI, Microsoft, and Google. The open-source program is called Code Llama, and its launch is imminent, according to The Information. (The Information)
OpenAI is testing GPT-4 for content moderation
Using the language model to moderate online content could really help alleviate the mental toll content moderation takes on humans. OpenAI says it’s seen some promising first results, although the tech does not outperform highly trained humans. A lot of big, open questions remain, such as whether the tool can be attuned to different cultures and pick up context and nuance. (OpenAI)
Google is working on an AI assistant that offers life advice
The generative AI tools could function as a life coach, offering up ideas, planning instructions, and tutoring tips. (The New York Times)
Two tech luminaries have quit their jobs to build AI systems inspired by bees
Sakana, a new AI research lab, draws inspiration from the animal kingdom. Founded by two prominent industry researchers and former Googlers, the company plans to make multiple smaller AI models that work together, the idea being that a “swarm” of programs could be as powerful as a single large AI model. (Bloomberg)